Unleashing the Power of Data Analytics
- Ruhi Parveen
- Feb 6, 2024
- 3 min read
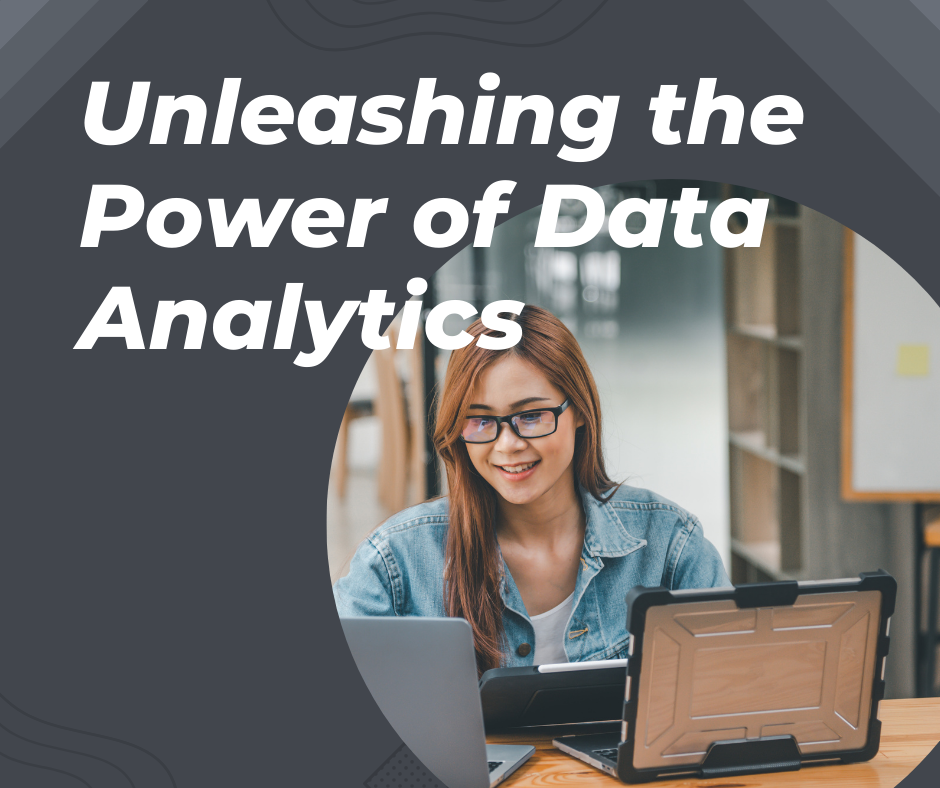
Introduction:
In today's digital age, data is being generated at an unprecedented rate across various sectors and industries. From online transactions to social media interactions, the sheer volume of data available is staggering. However, the real challenge lies in deriving meaningful insights from this vast amount of information. This is where data analytics comes into play. Data analytics is the process of examining data sets to uncover patterns, trends, and insights that can be used to make informed decisions. In this article, we will delve into the world of data analytics, exploring its significance, techniques, and applications across different domains.
Understanding Data Analytics:
At its core, data analytics involves the use of various tools and techniques to analyze data and extract valuable insights. These insights can range from identifying customer preferences to optimizing business processes. Data analytics can be broadly categorized into descriptive, diagnostic, predictive, and prescriptive analytics.
Descriptive analytics focuses on summarizing historical data to understand what has happened in the past. Diagnostic analytics aims to identify the reasons behind certain events or trends by digging deeper into the data. Predictive analytics utilizes statistical algorithms and machine learning techniques to forecast future outcomes based on historical data. Finally, prescriptive analytics goes a step further by recommending actions to optimize outcomes based on predictive models.
Techniques of Data Analytics:
Data analytics encompasses a wide range of techniques, each suited for different types of data and analytical objectives. Some of the commonly used techniques include:
Statistical Analysis: Statistical methods such as regression analysis, hypothesis testing, and clustering are used to analyze numerical data and identify relationships between variables.
Machine Learning: Machine learning algorithms enable computers to learn from data and make predictions or decisions without being explicitly programmed. These algorithms include decision trees, neural networks, and support vector machines.
Data Mining: Data mining techniques are used to discover patterns and relationships within large datasets. This includes association rule mining, classification, and clustering.
Text Analytics: Text analytics involves extracting insights from unstructured textual data, such as customer reviews, social media posts, and emails. Natural language processing (NLP) techniques are commonly used for sentiment analysis, topic modeling, and entity recognition.
Visualization: Data visualization techniques help in presenting complex data in a visual format, making it easier to interpret and analyze. This includes charts, graphs, heatmaps, and interactive dashboards.
Applications of Data Analytics:
Data analytics has numerous applications across various industries, revolutionizing how businesses operate and make decisions. Some of the key applications include:
Marketing and Sales: Data analytics is used to analyze customer behavior, segment markets, and personalize marketing campaigns. By understanding customer preferences and purchase patterns, businesses can target their offerings more effectively and improve sales.
Healthcare: In healthcare, data analytics is used for clinical decision-making, disease surveillance, and patient monitoring. Predictive analytics can help identify patients at risk of developing certain conditions, allowing for early intervention and preventive care.
Finance: In the finance industry, data analytics is used for risk management, fraud detection, and algorithmic trading. By analyzing market trends and customer behavior, financial institutions can make better investment decisions and mitigate risks.
Manufacturing: Data analytics is used in manufacturing for predictive maintenance, quality control, and supply chain optimization. By analyzing sensor data from equipment and production processes, manufacturers can detect anomalies and optimize workflows to improve efficiency and reduce downtime.
Smart Cities: Data analytics plays a crucial role in building smart cities by optimizing transportation systems, energy usage, and public services. By analyzing data from sensors and IoT devices, city planners can make data-driven decisions to improve urban infrastructure and quality of life.
Challenges and Future Trends:
Despite its immense potential, data analytics also poses certain challenges, including data privacy concerns, data quality issues, and the need for skilled professionals. However, with advancements in technology and the proliferation of data-driven decision-making, the future of data analytics looks promising.
Some of the emerging trends in data analytics include the use of artificial intelligence (AI) and machine learning for automation and predictive modeling, the integration of big data and IoT for real-time analytics, and the adoption of cloud-based analytics platforms for scalability and flexibility.
Conclusion:
In conclusion, data analytics has emerged as a powerful tool for extracting insights from data and driving informed decision-making across various industries. By leveraging advanced techniques and technologies, businesses can gain a competitive edge, improve operational efficiency, and enhance customer satisfaction. However, to fully realize the benefits of data analytics, organizations must invest in infrastructure, talent, and data governance frameworks. As we continue to generate and collect vast amounts of data, the role of data analytics will only become more critical in shaping the future of business and society. Those interested in delving deeper into this field can explore Data Analytics courses in Lucknow, Delhi, Noida, and all major cities in India to acquire the necessary skills and knowledge for leveraging data effectively. Also read: Insights Unleashed: A Guide to Profound Data Analytics
Comentários