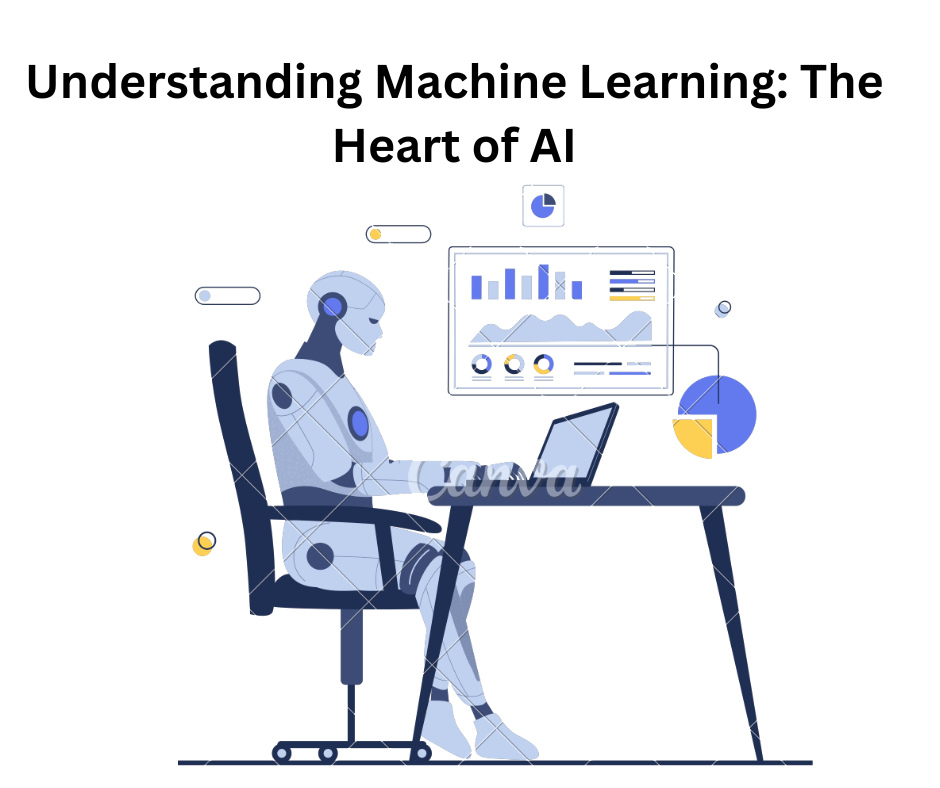
Machine learning is a transformative technology that lies at the core of artificial intelligence (AI). It has revolutionized various industries by enabling machines to learn from data, make predictions, and improve their performance over time. In this article, we will explore what machine learning is, how it works, its types, real-world applications, and its future potential.
What is Machine Learning?
Machine learning is a subset of artificial intelligence that focuses on the development of algorithms and models that allow computers to learn from and make decisions based on data. Instead of being explicitly programmed to perform a specific task, machine learning models are trained on large datasets and can identify patterns, make predictions, and improve their accuracy over time.
Key Concepts in Machine Learning
Data: The foundation of machine learning is data. The quality and quantity of data significantly impact the performance of a machine learning model.
Algorithms: Algorithms are the mathematical rules that guide the machine learning process. They help the model learn from data and make predictions.
Model: It is a mathematical representation of the patterns found in the data, which can be used to make predictions on new, unseen data.
Training: Training is the process of feeding data into the machine learning algorithm to help it learn the patterns in the data.
Prediction: Once the model is trained, it can be used to make predictions or decisions based on new data.
Evaluation: Evaluation involves testing the model's accuracy and performance using a separate dataset to ensure it can generalize well to new data.
How Does Machine Learning Work?
Machine learning uses algorithms to find patterns in data and make predictions based on those patterns. The process can be broken down into several key steps:
1. Data Collection
The first step in machine learning is to gather data.The quality and relevance of the data are crucial for building an effective machine learning model.
2. Data Preprocessing
This involves removing missing or irrelevant data, handling outliers, and transforming the data into a format suitable for the machine learning algorithm.
3. Choosing a Model
The next step is to choose a machine learning model. There are different types of models, each suitable for different types of data and tasks. The choice of model depends on the problem you are trying to solve.
4. Training the Model
The chosen model is then trained with the cleaned and prepared data.During training, the model learns to recognize patterns and relationships in the data. This process involves tweaking the model’s settings to reduce the gap between its predictions and the actual results.
5. Evaluation and Tuning
After training, the model is tested on a different set of data to see how well it performs. If the model's accuracy is not satisfactory, it may need to be tuned or retrained with more data or different parameters.
6. Making Predictions
Once the model is trained and tested, you can use it to make predictions on new data.These predictions can be used to inform decisions, automate processes, or provide insights.
Types of Machine Learning
1. Supervised Learning
In supervised learning, the model is trained on a labeled dataset, where each data point is associated with a known outcome.Supervised learning is commonly used in tasks like classification (e.g., spam detection) and regression (e.g., predicting house prices).
2. Unsupervised Learning
Unsupervised learning involves training a model on data without labeled outcomes. The goal is to identify patterns or groupings in the data. Common applications of unsupervised learning include clustering (e.g., customer segmentation) and dimensionality reduction (e.g., principal component analysis).
3. Reinforcement Learning
Reinforcement learning is a type of machine learning where an agent learns to make decisions by interacting with an environment. The agent receives rewards or penalties based on its actions and uses this feedback to improve its performance over time. Reinforcement learning is often used in robotics, gaming, and autonomous systems.
Real-World Applications of Machine Learning
Machine learning has a wide range of applications across various industries, transforming the way businesses operate and providing innovative solutions to complex problems.
1. Healthcare
Machine learning is being used in healthcare to improve diagnostics, personalize treatment plans, and predict patient outcomes. For example, machine learning models can analyze medical images to detect diseases like cancer with high accuracy.
2. Finance
In the finance industry, machine learning is used for fraud detection, algorithmic trading, risk management, and credit scoring. Machine learning models can analyze large volumes of financial data to identify fraudulent transactions or predict market trends.
3. E-commerce
E-commerce platforms use machine learning to provide personalized recommendations, optimize pricing strategies, and enhance customer service. By analyzing customer behavior and preferences, machine learning models can suggest products that users are likely to purchase.
4. Autonomous Vehicles
Machine learning is a key technology behind autonomous vehicles. These vehicles use machine learning algorithms to process sensor data, recognize objects, and make real-time decisions to navigate safely.
5. Natural Language Processing (NLP)
Machine learning is at the heart of natural language processing, enabling computers to understand and generate human language. Applications of NLP include speech recognition, machine translation, sentiment analysis, and chatbots.
The Future of Machine Learning
Machine learning is a rapidly evolving field with enormous potential. As technology advances, we can expect machine learning to become even more integrated into our daily lives and drive innovation across various sectors.
1. Improved Accuracy and Efficiency
As machine learning algorithms and models continue to improve, we can expect higher accuracy in predictions and more efficient processing of large datasets. This will lead to better decision-making and more effective automation of tasks.
2. Ethical and Responsible AI
With the increasing use of machine learning, there is a growing focus on the ethical implications of AI. Ensuring that machine learning models are fair, transparent, and free from bias is crucial for building trust and avoiding harmful outcomes.
3. Integration with Other Technologies
Machine learning will increasingly be integrated with other emerging technologies such as the Internet of Things (IoT), blockchain, and quantum computing. This integration will unlock new possibilities and create more powerful and versatile AI systems.
4. Democratization of AI
As machine learning tools and platforms become more accessible, more people and organizations will be able to leverage the power of AI. This democratization will lead to greater innovation and the development of new applications across various fields.
5. Lifelong Learning
Future machine learning systems may be designed to continuously learn and adapt over time. This concept, known as lifelong learning, would allow models to improve their performance as they encounter new data and environments.
Challenges in Machine Learning
Despite its potential, machine learning also faces several challenges that need to be addressed to ensure its continued success.
1. Data Quality and Availability
Ensuring that data is accurate, complete, and representative of the problem domain is crucial for building effective models.
2. Model Interpretability
As machine learning models become more complex, it becomes increasingly difficult to understand how they make decisions. Improving model interpretability is important for building trust and ensuring that models are used responsibly.
3. Bias and Fairness
Machine learning models can inadvertently learn biases present in the data, leading to unfair or discriminatory outcomes. Addressing bias and ensuring fairness in machine learning models is a critical challenge.
4. Scalability
As the volume of data continues to grow, machine learning models must be able to scale to handle large datasets efficiently. Developing scalable algorithms and infrastructure is essential for the future of machine learning.
5. Security and Privacy
Machine learning models can be vulnerable to adversarial attacks and privacy breaches. Ensuring the security and privacy of data and models is a significant concern in the deployment of machine learning systems.
Conclusion
Machine learning is the driving force behind many of the advancements in artificial intelligence today. It has the potential to transform industries, improve decision-making, and create new opportunities for innovation. As we continue to develop and refine machine learning technologies, it is important to address the challenges and ethical considerations that come with it. By enrolling in the Best Artificial Intelligence Course in Noida, Delhi, Mumbai, Indore, and other parts of India, individuals can gain the skills and knowledge necessary to harness the full potential of machine learning and contribute to building a better and more intelligent future.
Comentários