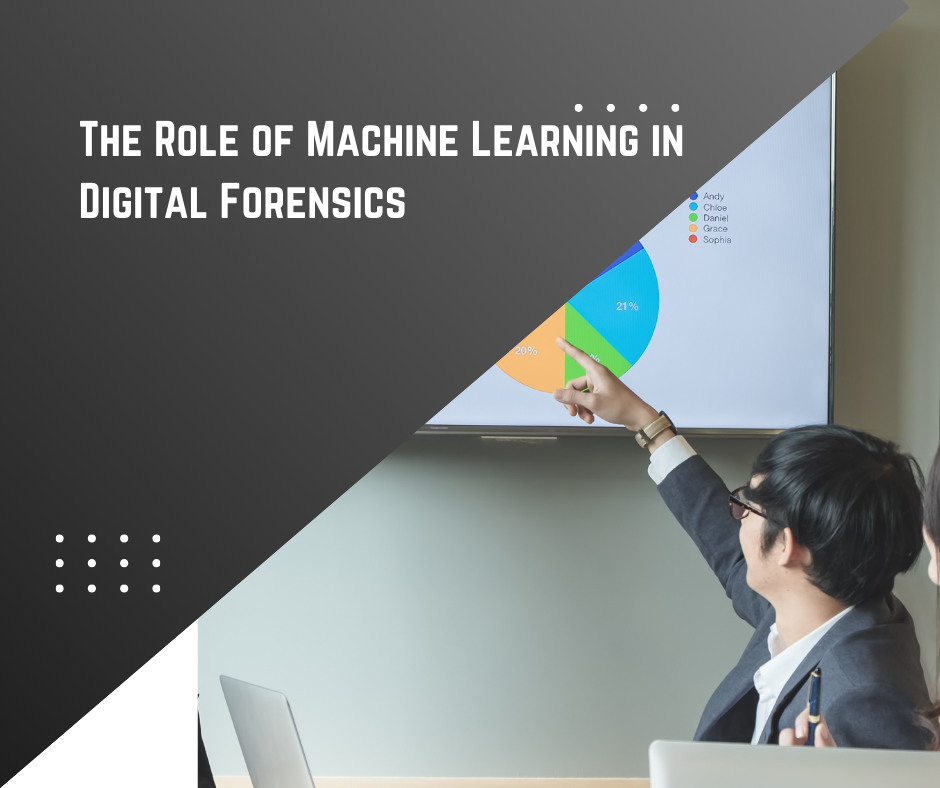
Introduction
Digital forensics involves the investigation of digital devices to uncover, analyze, and report electronic data that can be used in legal proceedings. With the exponential growth of data and the increasing sophistication of cybercrimes, traditional methods of digital forensics are becoming less effective. Machine learning (ML) offers innovative solutions to enhance the efficiency and accuracy of digital forensics. This article explores the role of machine learning in digital forensics, highlighting its benefits, applications, and challenges.
What is Machine Learning?
Machine learning is a branch of artificial intelligence (AI) that focuses on creating systems that can learn from data and make decisions with little human input. It involves designing algorithms that can examine data, recognize patterns, and make predictions or decisions based on what they have learned. In digital forensics, machine learning can automate the analysis of large datasets, identify anomalies, and provide insights that would be difficult for humans to detect.
Benefits of Machine Learning in Digital Forensics
1. Increased Efficiency
Machine learning algorithms can process vast amounts of data much faster than human investigators. This increased efficiency allows forensic analysts to focus on the most relevant data, speeding up investigations and reducing the time needed to reach conclusions.
2. Enhanced Accuracy
Machine learning models can identify patterns and anomalies that might be overlooked by human analysts. This enhances the accuracy of forensic investigations, ensuring that critical evidence is not missed.
3. Automation of Repetitive Tasks
Many tasks in digital forensics are repetitive and time-consuming. Machine learning can automate these tasks, such as data classification and sorting, freeing up investigators to focus on more complex aspects of the investigation.
4. Detection of Advanced Threats
CCybercriminals are always coming up with new methods to avoid getting caught. Machine learning models can adapt to these evolving threats, identifying new types of malware, phishing attacks, and other cyber threats that traditional methods might miss.
Applications of Machine Learning in Digital Forensics
1. Malware Detection and Analysis
Machine learning algorithms can analyze files and network traffic to detect and classify malware. By learning from known malware samples, these algorithms can identify new, previously unknown malware based on its behavior and characteristics.
2. Anomaly Detection
Machine learning can be used to detect anomalies in network traffic, user behavior, and system logs. Anomalies can indicate potential security breaches, insider threats, or other malicious activities that require further investigation.
3. Data Recovery
In cases where data has been intentionally deleted or corrupted, machine learning techniques can assist in data recovery. Algorithms can analyze the remnants of files and reconstruct them, providing valuable evidence that might otherwise be lost.
4. User Behavior Analysis
Machine learning can analyze patterns in user behavior to identify unusual activities. This can help in detecting unauthorized access, insider threats, and other security incidents that involve user accounts.
5. Image and Video Analysis
Digital forensics often involves the analysis of multimedia files. Machine learning models can automate the analysis of images and videos, identifying faces, objects, and other relevant features that can be used as evidence.
Challenges of Implementing Machine Learning in Digital Forensics
1. Data Quality and Quantity
Machine learning models require large amounts of high-quality data to train effectively. In digital forensics, obtaining sufficient data that is accurately labeled can be challenging.
2. Model Interpretability
Machine learning models, especially deep learning models, can be complex and difficult to interpret. In legal contexts, it is essential to understand how a model reached its conclusions to ensure the evidence is admissible in court.
3. Constantly Evolving Threats
Cyber threats are constantly evolving, and machine learning models need to be regularly updated to stay effective. This requires ongoing research and development to keep up with new attack techniques.
4. Resource Intensive
Training and deploying machine learning models can be resource-intensive, requiring significant computational power and expertise. Smaller organizations may find it challenging to implement and maintain these systems.
5. Bias and Fairness
Machine learning models can be biased based on the data they are trained on. In digital forensics, this can lead to unfair outcomes or incorrect conclusions if the models are not carefully designed and tested.
Future of Machine Learning in Digital Forensics
1. Integration with Artificial Intelligence
The integration of machine learning with other AI technologies, such as natural language processing and computer vision, will further enhance digital forensics capabilities. This will enable more comprehensive analysis of diverse data types, from text and speech to images and videos.
2. Real-Time Analysis
Advancements in machine learning will enable real-time analysis of digital data, allowing forensic investigators to detect and respond to incidents as they occur. This will be particularly valuable in preventing data breaches and minimizing damage from cyber attacks.
3. Improved Collaboration Tools
Machine learning will facilitate improved collaboration among forensic investigators, law enforcement agencies, and other stakeholders. Shared platforms powered by machine learning can help streamline the exchange of information and insights, making investigations more efficient.
4. Ethical and Legal Considerations
As machine learning becomes more prevalent in digital forensics, there will be increased focus on ethical and legal considerations. Ensuring that machine learning models are transparent, fair, and accountable will be crucial in maintaining public trust and ensuring the integrity of forensic investigations.
Conclusion
Machine learning is revolutionizing the field of digital forensics by enhancing the efficiency, accuracy, and scope of investigations. Its applications in malware detection, anomaly detection, data recovery, user behavior analysis, and multimedia analysis are transforming how digital evidence is analyzed and utilized. However, challenges such as data quality, model interpretability, evolving threats, resource intensity, and bias must be addressed to fully realize the potential of machine learning in digital forensics. As technology advances, the integration of machine learning with other AI technologies and the focus on real-time analysis and ethical considerations will shape the future of digital forensics, making it more effective in combating cybercrime and securing digital evidence. For those looking to gain expertise in this transformative field, Machine Learning Training in Noida, Delhi, Mumbai, Indore, and other parts of India provides a comprehensive foundation, equipping professionals with the necessary skills to tackle these emerging challenges and opportunities.
Comments