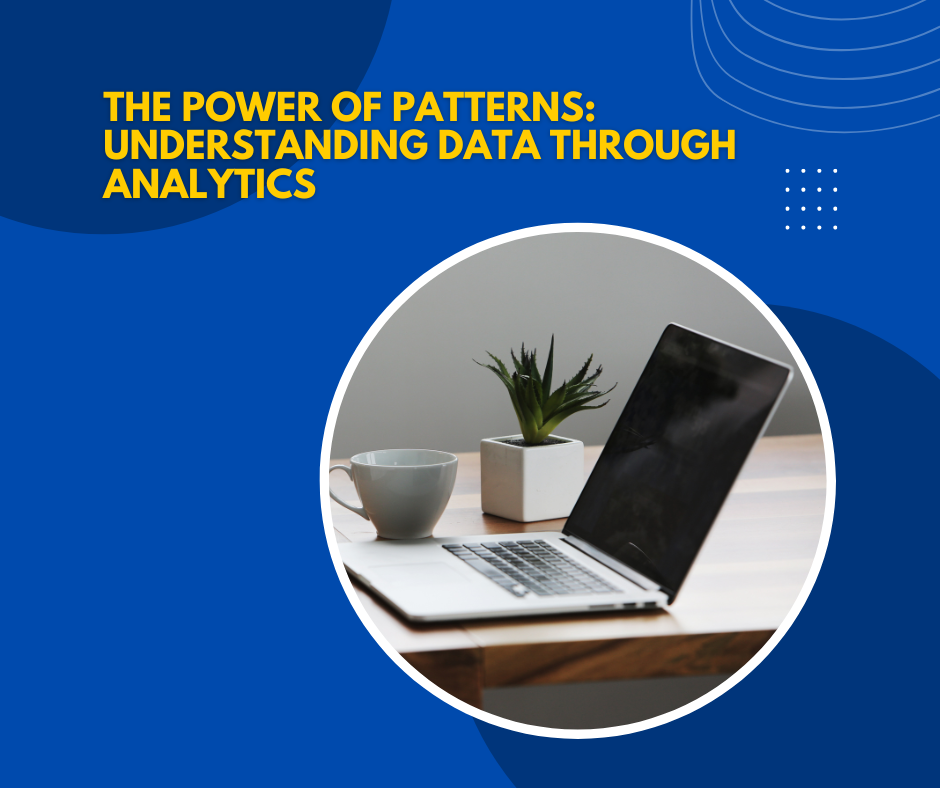
In the modern age, data has become one of the most valuable resources for organisations, helping them make informed decisions, optimise operations, and stay ahead of the competition. However, data on its own can be overwhelming and unmanageable. To harness its true potential, businesses must analyse it to uncover meaningful patterns and insights. This process of deriving value from data is known as data analytics. In this guide, we will explore how patterns in data can provide powerful insights and why understanding these patterns is essential for successful data-driven decision-making.
What is Data Analytics?
Data analytics is the science of examining raw data to find trends and draw conclusions about the information it contains. This practice involves using a range of tools and techniques to process data and uncover patterns that can guide strategic decisions. Companies use data analytics to understand market trends, customer preferences, operational performance, and much more.
Data analytics four main types:
Descriptive Analytics: Focuses on what has happened in the past. It provides historical data summaries and metrics.
Diagnostic Analytics: Helps explain why something happened by identifying patterns and relationships in data.
Predictive Analytics: Uses historical data and statistical techniques to predict future outcomes.
Prescriptive Analytics: Suggests actions to take based on the predictions and analyses.
The Importance of Patterns in Data
Patterns are recurring relationships, trends, or structures found in data. Identifying these patterns is key to understanding the behaviour of systems, processes, or groups of individuals. By recognizing patterns, businesses can make predictions, solve problems, and improve their operations.
1. Patterns Reveal Insights
Patterns are powerful because they reveal hidden relationships in the data that would otherwise be difficult to detect. For example, a retail business might analyse sales data and find a recurring pattern in customer purchases—such as increased sales during holidays. This insight helps the company plan promotions and stock inventory accordingly.
2. Patterns Help Predict Future Outcomes
One of the greatest benefits of discovering patterns is the ability to predict future outcomes. In healthcare, for instance, patient data may show recurring patterns that indicate certain risk factors for diseases. By recognizing these patterns early, healthcare providers can predict and prevent future health problems, leading to better patient care.
3. Patterns Enable Better Decision-Making
Once patterns are identified, organisations can use this information to make more informed and data-driven decisions. For example, a transportation company can analyse route data and find patterns that highlight inefficiencies in delivery times. With this knowledge, they can optimise routes to save time and costs.
4. Patterns Improve Efficiency
Patterns can reveal inefficiencies and areas for improvement. For instance, analysing manufacturing data might show a recurring pattern of machine downtime, leading the company to invest in maintenance solutions that reduce production interruptions.
Methods for Detecting Patterns in Data
There are several methods used in data analytics to detect patterns. These methods include statistical techniques, machine learning algorithms, and data visualisation tools.
1. Statistical Analysis
Statistical methods, such as regression analysis, correlation analysis, and clustering, are often used to detect patterns in data. These methods rely on mathematical models to identify relationships between variables and uncover trends.
Regression Analysis: Helps understand the relationship between dependent and independent variables.
Correlation Analysis: Measures the strength and direction of relationships between two variables.
Clustering: Groups similar data points together to identify patterns.
2. Machine Learning
Machine learning (ML) algorithms are a powerful tool for discovering complex patterns in large datasets. ML algorithms can automatically learn from data and make predictions without being explicitly programmed for a specific task.
Supervised Learning: This involves training an algorithm on a labelled dataset, where the desired output is known, to recognize patterns and predict future outcomes.
Unsupervised Learning: This method finds hidden patterns in data without labelled outcomes, such as clustering similar customers based on their purchasing behaviours.
3. Data Visualization
Visualisation tools such as graphs, charts, and heatmaps help to represent data patterns in a way that is easy to understand. A well-designed visualisation can reveal trends and correlations that might not be immediately obvious in raw data.
Bar Charts: Compare categorical data, showing trends or changes over time.
Scatter Plots: Reveal relationships between two variables.
Heatmaps: Visualise the magnitude of a phenomenon by colour-coding different values.
Applications of Data Analytics in Various Industries
The power of patterns in data analytics extends to a wide range of industries. Here are some key examples of how different sectors benefit from analysing data to find patterns.
1. Retail
Retailers use data analytics to understand customer preferences, buying patterns, and market trends. By analysing data, businesses can personalise marketing campaigns, optimise inventory management, and improve customer satisfaction.
Example: A retail company may analyse purchase histories to find that customers who buy a certain product are likely to purchase a complimentary item within a month. They can use this pattern to send targeted marketing messages.
2. Healthcare
In healthcare, data analytics can help predict disease outbreaks, improve patient care, and optimise hospital operations. Patterns in patient data, such as recurring symptoms, can help doctors diagnose conditions more accurately and provide better treatment plans.
Example: By analysing medical records, healthcare providers may detect patterns indicating that certain patients are at high risk for developing chronic illnesses, allowing for early intervention.
3. Finance
Financial institutions use data analytics to detect fraud, assess risk, and personalise services for customers. Identifying patterns in transaction data can help prevent fraudulent activities and improve the accuracy of credit scoring.
Example: A bank might detect a pattern of unusual transactions on a customer’s account, leading to a quick response to prevent potential fraud.
4. Manufacturing
Manufacturers use data analytics to optimise production processes, reduce waste, and enhance product quality. Patterns in machine data can indicate when maintenance is needed, preventing costly downtime and ensuring efficient operations.
Example: Predictive analytics can identify patterns in machine wear and tear, allowing manufacturers to schedule maintenance before breakdowns occur.
5. Marketing
Marketing teams use data analytics to understand customer behaviour, measure campaign effectiveness, and optimise strategies. By analysing customer data, marketers can identify segments of audiences that respond best to specific types of messaging and tailor their outreach accordingly.
Example: A marketing team may find a pattern where customers who engage with email campaigns are more likely to purchase during flash sales. They can use this insight to schedule timely promotional emails.
Challenges in Detecting Patterns
While patterns can provide valuable insights, detecting them is not always easy. Several challenges may arise when analysing data, including:
1. Data Quality
If the data is incomplete, inconsistent, or inaccurate, it can be difficult to identify meaningful patterns. Ensuring high-quality data is critical for effective analysis.
2. Data Overload
With vast amounts of data generated every day, it can be overwhelming to analyse all of it. Data overload can make it difficult to detect relevant patterns, making it essential to use appropriate tools and techniques.
3. Bias in Data
Biases in data can lead to misleading patterns and inaccurate conclusions. It’s important to use diverse datasets and apply proper statistical methods to avoid bias.
4. Complexity of Patterns
Sometimes, patterns in data are complex and not easily recognizable. This is where advanced machine learning algorithms and statistical models come in, helping to uncover hidden patterns that humans might miss.
The Future of Data Analytics
As data volume continues to expand, the significance of data analytics will only grow. In the future, advancements in artificial intelligence (AI) and machine learning will allow organisations to detect even more complex patterns in real-time. Additionally, industries that adopt data-driven strategies will be able to gain a competitive edge by making more accurate predictions and more informed decisions.
Conclusion
Data analytics holds immense power when it comes to understanding patterns in data. These patterns help organizations predict future outcomes, make better decisions, and improve efficiency. From retail to healthcare, finance to manufacturing, every industry can benefit from recognizing and acting on the insights hidden in their data. As data continues to grow in importance, learning how to detect and interpret patterns will be an essential skill for businesses and individuals alike. For those looking to enhance their skills, the Data Analytics Training Institute in Delhi, Noida, Mumbai, Indore, and other parts of India offers valuable resources to master this critical field.
Comments