The Future of Machine Learning: Trends for 2025
- Ruhi Parveen
- Jun 28, 2024
- 4 min read
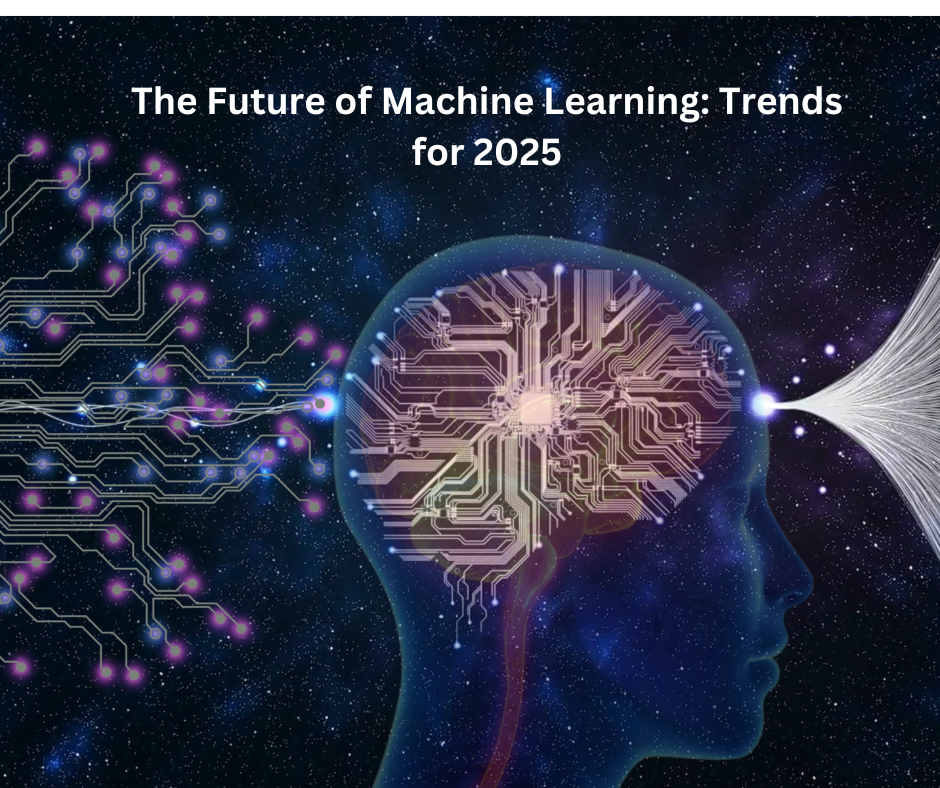
Machine Learning (ML) has been one of the most transformative technologies in recent years, driving innovation across various industries. As we look toward 2025, several emerging trends and predictions will shape the future of ML. This article explores these trends, offering insights into what we can expect in the coming years.
1. Increased Integration of AI and ML in Everyday Applications
Consumer Applications
By 2025, AI and ML will be more deeply integrated into everyday consumer applications. Virtual assistants like Siri, Alexa, and Google Assistant will become more intelligent and context-aware, providing more personalized and accurate responses. Smart home devices will utilize ML to automate and optimize household functions, such as energy management and security.
Business Applications
Businesses will increasingly adopt ML to enhance customer service, optimize operations, and improve decision-making. For example, customer support chatbots will become more sophisticated, capable of handling complex queries and providing human-like interactions. Predictive analytics will enable companies to anticipate market trends and make data-driven decisions.
2. Advancements in Natural Language Processing (NLP)
Conversational AI
Natural Language Processing (NLP) will see significant advancements, leading to more natural and engaging conversational AI. By 2025, virtual assistants and chatbots will understand and respond to human language with greater accuracy and nuance, making interactions more seamless and intuitive.
Multilingual Capabilities
NLP models will become more proficient in understanding and generating text in multiple languages, breaking down language barriers and enabling global communication. This will be particularly beneficial for multinational companies and platforms serving diverse user bases.
3. Edge Computing and ML
Decentralized Processing
Edge computing will play a crucial role in the future of ML by bringing data processing closer to the source of data generation. This will reduce latency and bandwidth usage, enabling real-time decision-making for applications like autonomous vehicles, smart cities, and industrial IoT.
Improved Privacy and Security
Processing data at the edge also enhances privacy and security by minimizing the need to transmit sensitive data to centralized servers. ML models will be deployed on edge devices, ensuring that personal and proprietary information remains protected.
4. Federated Learning
Collaborative Model Training
Federated learning will gain traction as a method for training ML models across multiple decentralized devices without sharing raw data. This approach allows organizations to collaboratively improve model accuracy while preserving data privacy. For instance, healthcare institutions can share insights from patient data without compromising patient confidentiality.
Enhanced Data Privacy
Federated learning will address growing concerns about data privacy and compliance with regulations like GDPR and CCPA. By keeping data localized and only sharing model updates, organizations can ensure that sensitive information is never exposed.
5. Automated Machine Learning (AutoML)
Simplified ML Model Development
AutoML will democratize machine learning by making it accessible to non-experts. Automated tools will handle tasks such as data preprocessing, feature selection, model selection, and hyperparameter tuning, enabling users to build high-quality models with minimal effort.
Accelerated Innovation
With AutoML, businesses can quickly prototype and deploy ML models, accelerating the pace of innovation. This will be particularly valuable for small and medium-sized enterprises (SMEs) that lack extensive data science resources.
6. Explainable AI (XAI)
Transparency in ML Models
As ML models become more complex, there will be a growing demand for transparency and interpretability. Explainable AI (XAI) techniques will provide insights into how models make decisions, allowing users to understand and trust the outcomes.
Regulatory Compliance
Explainable AI will also be critical for regulatory compliance in industries like finance and healthcare. Organizations will need to demonstrate that their ML models are fair, unbiased, and accountable, ensuring adherence to ethical standards and regulations.
7. Ethical AI and Responsible ML
Bias Mitigation
Addressing bias in ML models will be a top priority by 2025. Researchers and practitioners will develop more sophisticated techniques to identify and mitigate bias, ensuring that AI systems are fair and equitable.
Ethical Guidelines and Frameworks
Industry standards and ethical guidelines for AI and ML will become more prevalent. Organizations will adopt frameworks to ensure responsible AI practices, covering aspects such as transparency, accountability, and inclusivity.
8. Quantum Machine Learning
Enhanced Computational Power
Quantum computing has the potential to revolutionize machine learning by providing exponentially greater computational power. By 2025, we can expect early-stage applications of quantum ML that solve complex problems more efficiently than classical computers.
Research and Development
Ongoing research and development in quantum ML will pave the way for breakthroughs in fields like cryptography, drug discovery, and optimization problems. Quantum algorithms will enable new capabilities that were previously infeasible with traditional computing.
9. ML for Climate Change and Sustainability
Environmental Monitoring
Machine learning will play a vital role in addressing climate change and promoting sustainability. ML models will analyze satellite imagery and sensor data to monitor environmental changes, track deforestation, and predict natural disasters.
Sustainable Practices
Industries will leverage ML to optimize resource usage and reduce waste. For example, agriculture can benefit from precision farming techniques that use ML to optimize water and fertilizer usage, while the energy sector can improve grid management and renewable energy integration.
10. Collaboration Between Humans and AI
Augmented Intelligence
The future of ML will see a shift towards augmented intelligence, where humans and AI work collaboratively to achieve better outcomes. AI will assist humans in decision-making, providing insights and recommendations while leaving the final judgment to human expertise.
Enhanced Human-Machine Interaction
Advancements in ML will improve human-machine interaction, making AI systems more intuitive and user-friendly. This will enable users to interact with AI in a more natural and efficient manner, enhancing productivity and user satisfaction.
Conclusion
The future of machine learning promises to be exciting and transformative, with numerous trends and advancements shaping the landscape by 2025. Increased integration of AI in everyday applications, advancements in NLP, the rise of edge computing and federated learning, the democratization of ML through AutoML, and the emphasis on ethical AI are just a few of the key trends to watch. For those interested in staying ahead in this evolving field, pursuing the Best Data Analytics Training in Noida, Delhi, Nagpur, Mumbai, Indore, and other parts of India can provide a significant advantage.
As machine learning continues to evolve, it will bring about new opportunities and challenges. Organizations and individuals must stay informed and adapt to these changes to harness the full potential of ML and drive positive impact across various sectors. By embracing these trends and fostering a culture of innovation and responsibility, we can look forward to a future where machine learning enhances our lives and addresses some of the most pressing issues of our time.
Comments