The Future of Machine Learning: Trends and Predictions
- Ruhi Parveen
- Aug 27, 2024
- 6 min read
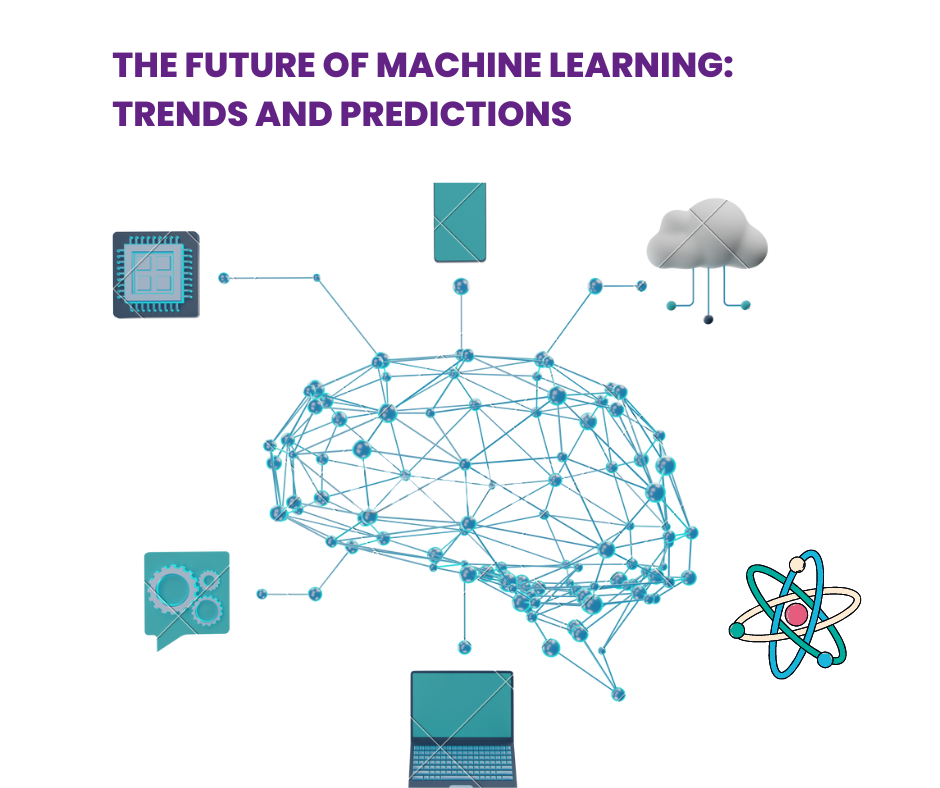
Machine Learning (ML) has rapidly evolved from a niche area within artificial intelligence to a mainstream technology driving innovation across various industries. As we move further into the digital age, ML continues to shape the future, offering transformative capabilities that are set to redefine the way businesses operate, how decisions are made, and how we interact with technology. This article explores the future of machine learning by examining key trends and making predictions about what lies ahead.
1. The Rise of Automated Machine Learning (AutoML)
Automated Machine Learning (AutoML) is poised to play a crucial role in democratizing ML by making it accessible to non-experts. AutoML tools allow users to automatically select models, optimize hyperparameters, and deploy models with minimal human intervention. This trend will enable more businesses to leverage ML without needing a team of data scientists, thereby accelerating the adoption of ML across various sectors.
1. Impact on Businesses
AutoML will significantly reduce the time and cost associated with building and deploying ML models. Companies can focus on solving business problems rather than getting bogged down in the technical complexities of ML. As a result, we can expect to see a surge in ML-powered solutions in industries like healthcare, finance, and retail.
2. Challenges and Considerations
While AutoML simplifies the process, it is not without challenges. The black-box nature of some AutoML systems can make it difficult to understand how decisions are made, which could lead to issues with transparency and trust. Therefore, developing explainable AutoML systems will be a critical area of focus.
2. Edge Computing and Machine Learning
Edge computing is another trend that will shape the future of ML. Instead of processing data in centralized cloud servers, edge computing allows data to be processed closer to where it is generated—on devices like smartphones, IoT devices, and sensors.
1. Benefits of Edge AI
The combination of edge computing and AI, often referred to as Edge AI, offers several advantages:
Reduced Latency: By processing data locally, Edge AI can deliver real-time insights and responses, which is crucial for applications like autonomous vehicles and smart cities.
Enhanced Privacy: Keeping data on the device reduces the need to transfer sensitive information over the internet, improving data security and privacy.
Cost Efficiency: Reducing the amount of data sent to the cloud can lower bandwidth and storage costs.
2. Predictions for Edge AI
As the capabilities of edge devices continue to grow, we will see more sophisticated ML models being deployed at the edge. This will enable smarter, more responsive applications in areas like healthcare, where real-time monitoring and analysis can save lives, and in manufacturing, where predictive maintenance can reduce downtime.
3. Ethical AI and Responsible Machine Learning
As ML becomes more pervasive, there is growing concern about the ethical implications of AI. Issues such as bias, fairness, transparency, and accountability are becoming increasingly important. The future of ML will be heavily influenced by how these ethical challenges are addressed.
1. Building Fair and Transparent Models
To build trust in AI systems, there is a need for greater transparency in how ML models make decisions. This includes developing techniques for interpreting and explaining ML models, as well as ensuring that these models are free from bias that could lead to unfair outcomes.
2. Regulations and Guidelines
Governments and organizations are likely to implement more regulations and guidelines to ensure the ethical use of AI. These regulations will require companies to take a proactive approach to auditing their ML models and ensuring they adhere to ethical standards.
3. The Role of AI Ethics Committees
We can expect the formation of AI ethics committees within organizations to oversee the development and deployment of ML systems. These committees will play a key role in ensuring that AI technologies are used responsibly and that their impact on society is positive.
4. Explainable AI (XAI)
Explainable AI (XAI) refers to methods and techniques that make the decision-making process of AI systems understandable to humans. As ML models become more complex, the need for XAI will grow, particularly in sectors where transparency is crucial, such as healthcare, finance, and law.
1. Importance of Explainability
Explainability is important for several reasons:
Compliance: In regulated industries, explainability is necessary to comply with legal requirements.
Improved Decision-Making: Understanding the factors that influence ML decisions can help improve the decision-making process and identify potential flaws in the models.
2. Future of XAI
The future of XAI will likely involve the development of new algorithms and tools that provide deeper insights into how ML models work. This could include visualizations, natural language explanations, and interactive systems that allow users to explore different scenarios and see how the model's decisions change.
5. The Integration of ML with Other Technologies
The future of ML will not be shaped by ML alone but by its integration with other emerging technologies. This convergence will unlock new possibilities and create powerful synergies.
1. ML and the Internet of Things (IoT)
The integration of ML with IoT will enable smarter, more autonomous systems. For example, in smart homes, ML algorithms can analyze data from various IoT devices to optimize energy usage, enhance security, and improve overall comfort.
2. ML and Blockchain
Combining ML with blockchain technology can enhance data security and integrity. Blockchain's decentralized nature can be used to create tamper-proof datasets for training ML models, which is particularly useful in industries like finance and healthcare where data integrity is critical.
3. ML and Quantum Computing
Quantum computing has the potential to revolutionize ML by providing the computational power needed to solve complex problems that are currently intractable. As quantum computing matures, we can expect to see breakthroughs in areas such as drug discovery, materials science, and cryptography, driven by quantum-enhanced ML algorithms.
6. AI in Healthcare
One of the most promising areas for the application of ML is healthcare. From diagnostics to personalized medicine, ML is transforming how we approach health and wellness.
1. Predictive Analytics in Healthcare
ML models can analyze vast amounts of data to predict health outcomes, identify at-risk patients, and recommend personalized treatment plans. This will lead to more proactive healthcare, where issues are addressed before they become serious problems.
2. Drug Discovery and Development
ML is accelerating the drug discovery process by identifying potential drug candidates faster than traditional methods. This can significantly reduce the time and cost of bringing new drugs to market, ultimately improving patient outcomes.
3. Robotics and Surgery
ML-powered robots are being used to assist in surgeries, providing greater precision and reducing the risk of human error. As these technologies advance, we will see more complex procedures being performed with the help of AI, improving patient safety and recovery times.
7. Machine Learning for Climate Change Mitigation
ML is also being leveraged to address one of the most pressing challenges of our time: climate change. By analyzing environmental data, ML can help us understand and mitigate the impact of climate change.
1. Energy Optimization
ML algorithms can optimize energy usage in buildings, transportation, and industrial processes, reducing carbon footprints and promoting sustainability. This is particularly important as the world shifts towards renewable energy sources.
2. Climate Modeling
ML is improving the accuracy of climate models by analyzing vast amounts of historical and real-time data. These models can predict the effects of climate change more accurately, helping policymakers make informed decisions.
3. Conservation Efforts
ML is being used in conservation efforts to monitor wildlife populations, track deforestation, and protect endangered species. By automating these processes, we can respond more quickly to environmental threats.
8. The Future Workforce and ML
The rise of ML will have a profound impact on the workforce, reshaping jobs and creating new opportunities.
1. Automation of Routine Tasks
ML will continue to automate routine and repetitive tasks, freeing up human workers to focus on more complex and creative endeavors. While this may lead to job displacement in some areas, it will also create new roles that require skills in AI and data analysis.
2. The Need for Continuous Learning
As ML becomes more integrated into various industries, there will be an increasing demand for workers with ML expertise. Continuous learning and upskilling will be essential for staying competitive in the job market.
3. Collaboration Between Humans and AI
The future workplace will likely involve close collaboration between humans and AI systems. Workers will need to develop new skills to effectively interact with and leverage AI tools, leading to a more dynamic and efficient workforce.
9. Conclusion
The future of machine learning is filled with exciting possibilities and challenges. From the rise of AutoML and Edge AI to the integration of ML with other cutting-edge technologies, machine learning is set to transform industries and society as a whole. To stay ahead, individuals and businesses should consider pursuing the Best Machine Learning Course in Delhi, Noida, Mumbai, Indore, and other parts of India. However, with these advancements comes the responsibility to address ethical concerns, ensure transparency, and prepare the workforce for a future where AI plays a central role. By staying informed about these trends and predictions, businesses and individuals can position themselves to thrive in the rapidly evolving landscape of machine learning.
Comments