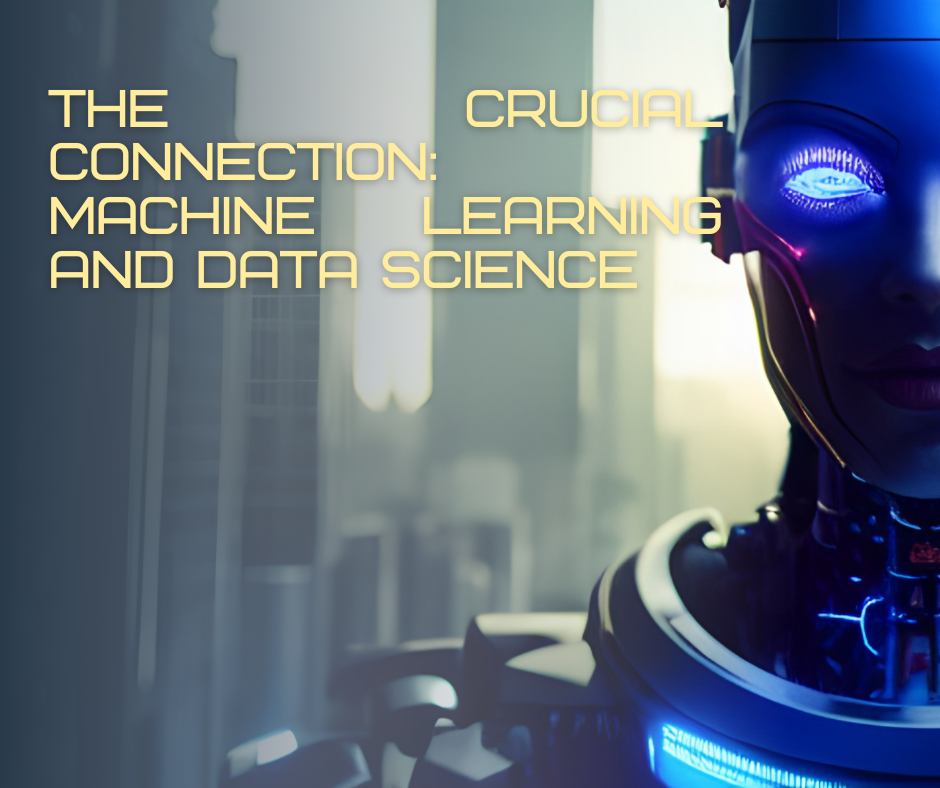
In the modern era, data has become the lifeblood of businesses, driving decision-making and strategy formulation. Data science and machine learning are two interconnected fields that play a crucial role in extracting insights from data and making predictions. In this article, we will explore the relationship between machine learning and data science, their significance, and how they are shaping the future of industries.
What is Data Science?
Data science is a multidisciplinary field that uses scientific methods, algorithms, and systems to extract knowledge and insights from structured and unstructured data. It combines principles from statistics, computer science, and domain knowledge to uncover hidden patterns, trends, and correlations in data.
The Role of Data Science:
Data science helps organizations make informed decisions by analyzing data and deriving actionable insights. It involves various stages such as data collection, cleaning, analysis, and visualization. Data scientists use tools like Python, R, and SQL to process and analyze data, and techniques like machine learning to build predictive models.
What is Machine Learning?
Machine learning is a subset of artificial intelligence that focuses on the development of algorithms and models that enable computers to learn from data and make predictions or decisions without being explicitly programmed. It uses statistical techniques to identify patterns in data and make intelligent decisions.
The Connection Between Machine Learning and Data Science:
Machine learning is an essential component of data science, as it provides the tools and techniques to analyze and interpret data. Data science, on the other hand, provides the framework and methodologies to collect, process, and analyze data, making it accessible for machine learning algorithms. Together, they form a powerful combination that enables organizations to derive valuable insights from data.
Applications of Machine Learning in Data Science:
Machine learning has various applications in data science, including:
Predictive Analytics: Machine learning algorithms can be used to make predictions based on historical data, such as forecasting sales or predicting customer churn.
Pattern Recognition: Machine learning can identify patterns and trends in data, helping organizations understand customer behavior or detect anomalies.
Natural Language Processing (NLP): Machine learning algorithms can process and analyze text data, enabling applications like chatbots and sentiment analysis.
Image Recognition: Machine learning models can analyze images to recognize objects, faces, or patterns, used in applications like medical imaging and autonomous vehicles.
Recommendation Systems: Machine learning algorithms can analyze user preferences and behavior to make personalized recommendations, as seen in streaming services and e-commerce platforms.
Challenges and Future Directions:
While machine learning and data science offer immense potential, they also face challenges such as data privacy, bias, and interpretability. Addressing these challenges will be crucial for the ethical and responsible use of these technologies.
The Future of Machine Learning and Data Science:
As technology advances, the future of machine learning and data science looks promising. Some key trends and developments shaping the field include:
Automated Machine Learning (AutoML): AutoML aims to automate the process of building machine learning models, making it accessible to non-experts and accelerating model development.
Explainable AI: There is a growing need for machine learning models to be explainable and interpretable, especially in critical applications like healthcare and finance, to ensure transparency and trust.
Edge Computing: Edge computing, which involves processing data closer to the source, is becoming increasingly important for real-time applications of machine learning, such as autonomous vehicles and IoT devices.
Federated Learning: Federated learning enables training machine learning models across multiple devices or servers without exchanging raw data, preserving privacy and security.
Ethical AI: As machine learning becomes more pervasive, there is a greater focus on ethical considerations, such as bias detection and mitigation, to ensure fair and responsible use of AI technologies.
Conclusion:
In conclusion, machine learning and data science are integral parts of the modern technological landscape, driving innovation and transforming industries. Their interconnection enables organizations to harness the power of data and make informed decisions. As these fields continue to evolve, addressing challenges and embracing new trends will be crucial for unlocking their full potential and shaping a more intelligent and data-driven future. For individuals looking to enter these fields, finding a reputable Data Science Training Institute in Delhi, Noida, Lucknow, Mumbai, and other cities in India can provide the necessary skills and knowledge to succeed in this dynamic industry.
Comments