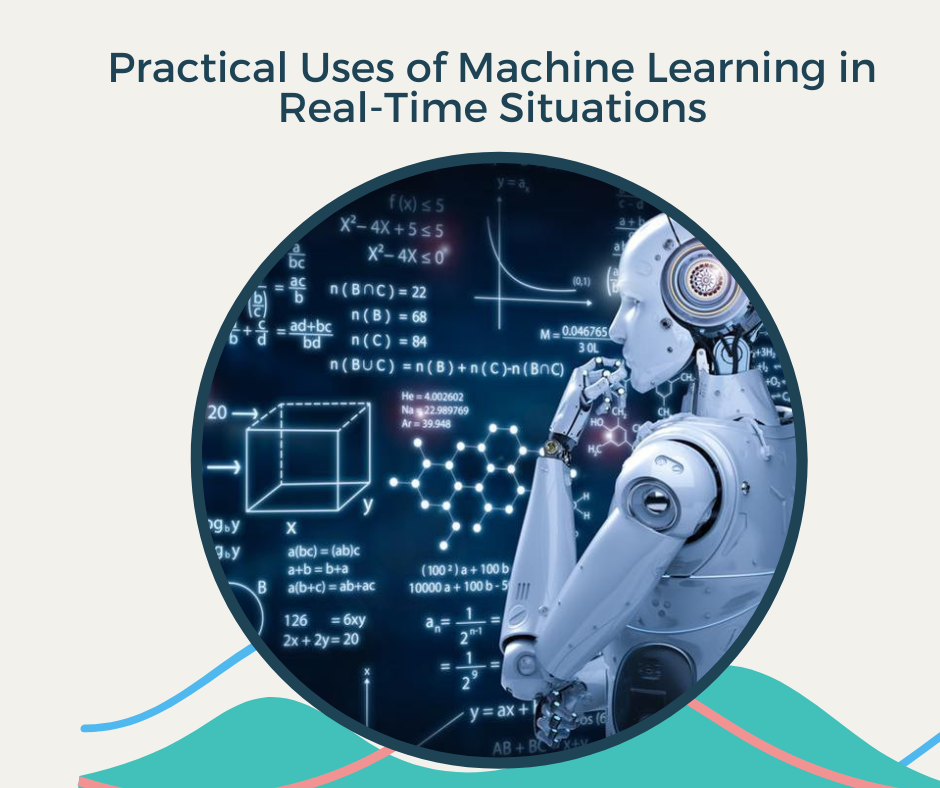
Machine learning (ML) has rapidly evolved from a niche area of computer science to a critical technology that underpins numerous real-time applications. Its ability to learn from data and make decisions or predictions has opened up endless possibilities. Below are some practical uses of machine learning in real-time situations that highlight its transformative power.
1. Healthcare and Medical Diagnosis
Machine learning has made significant strides in healthcare, particularly in medical diagnosis and treatment recommendations.
a. Early Disease Detection
Machine learning algorithms can analyze medical images, such as X-rays, MRIs, and CT scans, to detect diseases at an early stage. For instance, ML models are used to identify tumors, fractures, and other anomalies with high accuracy, sometimes surpassing human radiologists.
b. Personalized Treatment Plans
By analyzing patient data, including genetic information, lifestyle factors, and previous medical history, machine learning can recommend personalized treatment plans. This approach ensures that patients receive the most effective treatments tailored to their specific needs.
2. Finance and Fraud Detection
The financial sector relies heavily on machine learning for real-time analysis and decision-making.
a. Fraud Detection
Machine learning models are employed to detect fraudulent transactions by analyzing patterns and anomalies in transaction data. These models can identify suspicious activities in real-time, preventing financial losses and protecting users.
b. Algorithmic Trading
In stock markets, machine learning algorithms are used for algorithmic trading. These algorithms analyze market data in real-time to make trading decisions, optimizing for factors such as price, volume, and timing to maximize profits.
3. Customer Service and Support
Enhancing customer experience is another area where machine learning excels.
a. Chatbots and Virtual Assistants
Machine learning powers chatbots and virtual assistants like Siri, Alexa, and Google Assistant. These AI-driven tools can understand and respond to user queries in real-time, providing instant support and information.
b. Sentiment Analysis
By analyzing customer feedback and social media posts, machine learning models can determine customer sentiment. This helps businesses understand customer satisfaction and make necessary adjustments to improve their services.
4. Transportation and Logistics
a. Predictive Maintenance
ML algorithms predict equipment failures by analyzing data from sensors and historical maintenance records. This enables proactive maintenance, reducing downtime and costs associated with unexpected breakdowns.
b. Route Optimization
Logistics companies use machine learning to optimize delivery routes. By considering factors like traffic, weather, and road conditions, ML models suggest the most efficient routes, saving time and fuel.
5. Retail and E-commerce
The retail industry leverages machine learning to enhance customer experiences and streamline operations.
a. Personalized Recommendations
E-commerce platforms like Amazon and Netflix use machine learning to provide personalized product and content recommendations. These recommendations are based on user behavior, preferences, and browsing history, increasing customer engagement and sales.
b. Inventory Management
Machine learning models predict demand for products, helping retailers manage inventory more effectively. This ensures that popular items are always in stock while minimizing overstock and associated costs.
6. Smart Home Devices
The proliferation of smart home devices has been significantly boosted by machine learning.
a. Energy Management
Smart thermostats like Nest use machine learning to learn users' habits and adjust heating and cooling settings accordingly. This leads to optimized energy consumption and cost savings.
b. Home Security
Machine learning enhances home security systems by analyzing data from cameras and sensors. It can detect unusual activities, recognize familiar faces, and send real-time alerts to homeowners.
7. Natural Language Processing (NLP)
NLP, a subset of machine learning, focuses on the interaction between computers and human language.
a. Language Translation
Machine learning models like Google Translate can translate text from one language to another in real-time, facilitating communication across language barriers.
b. Speech Recognition
Speech recognition technology, powered by machine learning, enables applications like transcription services and voice-activated controls. These systems can convert spoken language into text and understand commands in real-time.
8. Autonomous Vehicles
Self-driving cars are one of the most advanced applications of machine learning.
a. Object Detection and Avoidance
Machine learning models process data from cameras, lidar, and other sensors to detect and avoid obstacles.
b. Route Planning
Autonomous vehicles use machine learning to plan routes in real-time, considering factors like traffic, road conditions, and construction. This ensures efficient and safe travel from point A to point B.
9. Cybersecurity
Machine learning enhances cybersecurity measures by identifying threats and vulnerabilities.
a. Threat Detection
ML models analyze network traffic and user behavior to detect potential security threats. They can identify patterns indicative of cyber attacks, such as malware or phishing attempts, and respond in real-time.
b. User Authentication
Machine learning improves user authentication processes by analyzing biometric data, such as fingerprints and facial recognition. This ensures secure and seamless access to systems and applications.
10. Agriculture
Machine learning is revolutionizing agriculture by optimizing various farming practices.
a. Crop Monitoring
Drones equipped with ML models monitor crop health by analyzing images and environmental data. This helps farmers detect diseases, pests, and nutrient deficiencies early.
b. Yield Prediction
Machine learning models predict crop yields by analyzing historical data, weather patterns, and soil conditions. This enables farmers to make informed decisions about planting and harvesting.
Conclusion
Machine learning's practical uses in real-time situations are vast and continually expanding. From healthcare and finance to customer service and agriculture, ML is transforming industries by providing intelligent, data-driven solutions. As technology advances, the potential for machine learning to further enhance real-time applications is immense, promising a future where intelligent systems are integral to our daily lives. For those looking to harness these advancements, Machine Learning Training in Noida, Delhi, Mumbai, Indore, and other parts of India offers valuable opportunities to gain the skills needed to be at the forefront of this exciting field.
Comments