Mastering A/B Testing and Experimentation in Data Science
- Ruhi Parveen
- Oct 8, 2024
- 4 min read
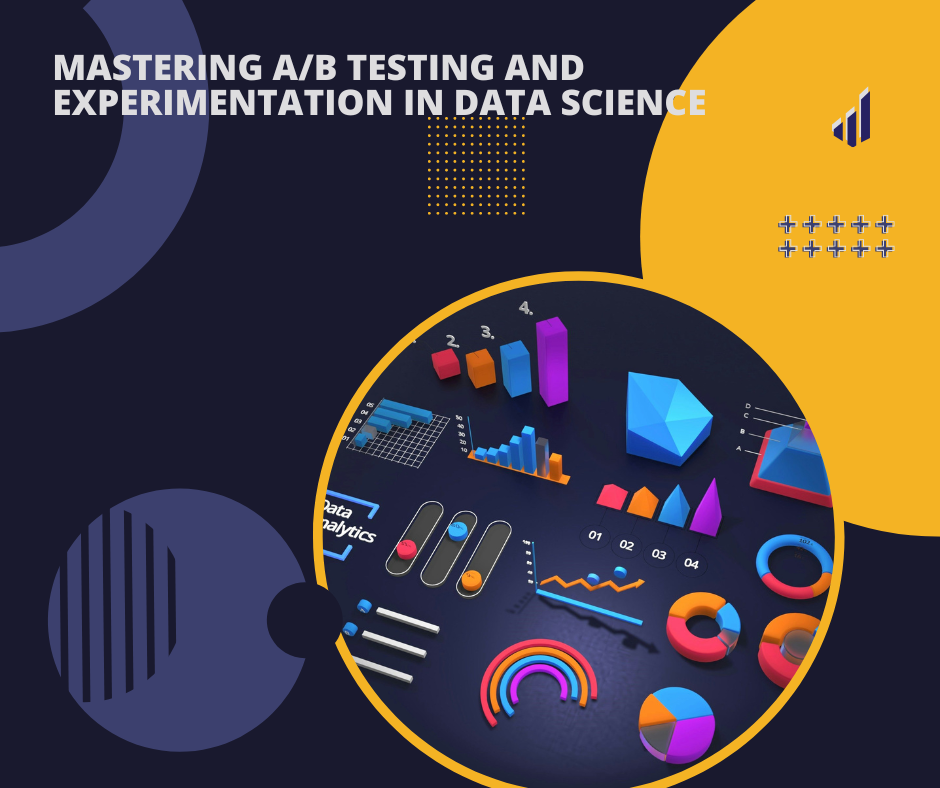
In the ever-evolving landscape of data science, making informed decisions is paramount. Whether you're optimising website conversions, refining product features, or enhancing marketing strategies, the power of data-driven insights cannot be overstated.At the core of data-driven decision-making is A/B testing and experimentation. This approach enables businesses to compare different versions of a variable to identify which one yields better results. In this comprehensive guide, we delve into the intricacies of A/B testing and experimentation, exploring its significance, methodologies, best practices, and real-world applications.
Understanding A/B Testing: The Foundation of Data-Driven Decision Making
A/B testing, also known as split testing, is a controlled experiment where two variants (A and B) are compared, typically by testing a subject's response to variant A against variant B. The goal is to identify which variant yields better results, such as higher conversion rates, increased engagement, or enhanced user satisfaction.
The Significance of A/B Testing:
Empirical Decision Making: A/B testing replaces intuition and guesswork with empirical evidence, enabling organizations to make data-driven decisions backed by statistical significance.
Continuous Improvement: By systematically testing variations, businesses can iteratively refine their products, services, and strategies to optimize performance over time.
Risk Mitigation: A/B testing allows for controlled experimentation, minimizing the risks associated with implementing changes based solely on assumptions or trends.
Customer-Centricity: By understanding customer preferences and behavior through experimentation, organizations can tailor their offerings to meet evolving needs effectively.
The Methodology of A/B Testing: From Hypothesis to Analysis
A successful A/B test follows a structured methodology, encompassing several key stages:
1. Define Objectives and Hypotheses:
Before conducting an A/B test, it's essential to clearly define the objectives and formulate hypotheses. What specific metrics are you trying to improve? What changes do you anticipate will yield better results?
2. Design Variants:
Develop distinct variations (A and B) based on the hypotheses, ensuring that each variant represents a single, isolated change. This could involve altering website layout, modifying product features, adjusting pricing strategies, or refining marketing messaging.
3. Randomized Allocation:
Randomly assign participants to each variant to ensure a representative sample and mitigate selection bias. Randomization helps ensure that any observed differences in outcomes are attributable to the variations being tested rather than external factors.
4. Implement Experiment:
Deploy the experiment, presenting each participant with either variant A or B based on the randomized allocation. It's crucial to maintain consistency in how the experiment is conducted to minimize confounding variables and ensure the validity of results.
5. Gather Data:
Collect relevant data points, such as conversion rates, click-through rates, or user engagement metrics, from both variants throughout the duration of the experiment. Sufficient sample size and duration are vital to obtain statistically significant results.
6. Statistical Analysis:
Apply appropriate statistical tests, such as t-tests or chi-square tests, to analyze the data and determine if the observed differences in outcomes between variants are statistically significant. This analysis helps ascertain whether the observed effects are likely due to the variations being tested or mere chance.
7. Draw Conclusions:
Based on the statistical analysis, draw conclusions regarding the performance of each variant relative to the defined objectives. Determine whether there's a clear winner or if further iterations or experiments are warranted.
Best Practices for Effective A/B Testing
While A/B testing offers valuable insights, executing experiments successfully requires adherence to best practices:
Focus on One Variable: Test one change at a time to isolate its impact accurately. Testing multiple variables simultaneously can muddy the results and make it challenging to discern causality.
Sufficient Sample Size: Ensure the experiment has a large enough sample size to detect meaningful differences with statistical significance. Small sample sizes can lead to unreliable results and erroneous conclusions.
Duration and Seasonality: Consider the duration of the experiment and account for any seasonal or temporal factors that may influence outcomes. Longer experiments help capture variations in behavior over time and reduce the risk of skewed results.
Segmentation and Personalization: Segment the audience based on relevant criteria, such as demographics or user behavior, to tailor experiments and derive more nuanced insights. Personalizing experiences can lead to more impactful optimizations.
Continuous Testing: A/B testing is an iterative process. Continuously test and refine variations to drive ongoing improvements and stay responsive to changing market dynamics.
Ethical Considerations: Ensure experiments adhere to ethical guidelines and respect user privacy. Transparent communication and informed consent are essential to maintaining trust and integrity.
Documentation and Collaboration: Document experiment details, methodologies, and results comprehensively to facilitate collaboration and knowledge sharing within the organization. Clear documentation fosters a culture of data-driven decision-making.
Real-World Applications of A/B Testing
A/B testing finds applications across various industries and domains, empowering organizations to optimize performance and enhance user experiences:
1. E-commerce and Retail:
E-commerce platforms leverage A/B testing to optimize product listings, pricing strategies, checkout processes, and promotional campaigns. Testing different variations of product images, descriptions, and pricing can significantly impact conversion rates and revenue.
2. Digital Marketing:
Marketers use A/B testing to refine email marketing campaigns, ad creatives, landing pages, and call-to-action buttons. By testing different messaging, visuals, and offers, marketers can identify the most effective strategies for driving engagement and conversions.
3. Software Development:
Software developers employ A/B testing to evaluate user interfaces, feature enhancements, and onboarding processes. Testing variations of UI elements, feature placements, and user workflows helps improve usability and customer satisfaction.
4. Content Publishing:
Publishers and content creators conduct A/B tests to optimize headlines, article layouts, and content formats. Testing different headline styles, imagery, and content lengths can influence reader engagement and retention.
5. Mobile App Optimization:
Mobile app developers utilize A/B testing to enhance user onboarding, navigation flows, and in-app messaging. Testing variations of app layouts, onboarding tutorials, and push notifications can drive user retention and app monetization.
Conclusion: Harnessing the Power of A/B Testing for Informed Decision Making
In the realm of data science, A/B testing and experimentation serve as indispensable tools for driving informed decision-making and continuous improvement. By systematically testing variations, analyzing outcomes, and iterating based on insights, organizations can optimize performance, enhance user experiences, and stay competitive in dynamic markets. Embracing the principles of A/B testing empowers businesses to unlock the full potential of their data and derive actionable insights that drive meaningful outcomes. As technology evolves and consumer preferences shift, mastering the art of A/B testing remains a cornerstone of data-driven success. Consider enrolling in a Data Science Training Course in Delhi, Noida, Lucknow, Mumbai, and other cities in India to enhance your skills and stay ahead in this field.
Read more: https://thepoliticus.com/advanced-tableau-for-data-science-and-business-intelligence/ Also read more: https://www.trangran.com/difference-between-business-intelligence-and-data-science/ Read more: https://www.herbaltricks.com/Articles-of-2024/unravelling-complexity-exploring-roles-and-responsibilities-data-scientist
Comments