Master Data Analytics: A Complete Guide for Beginners in 2025
- Ruhi Parveen
- Jan 11
- 5 min read
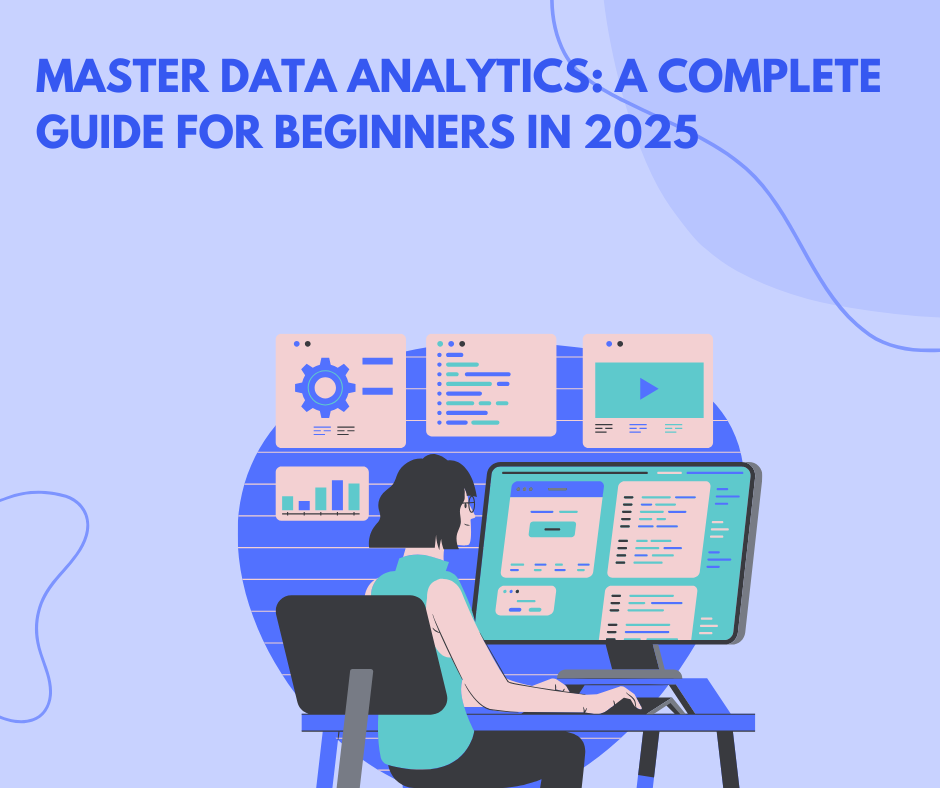
Data analytics has become one of the most important skills in today’s data-driven world. From business decisions to technology innovations, data plays a crucial role in shaping our understanding of the world. This complete guide is designed to help beginners in 2025 understand the fundamentals of data analytics, its key concepts, tools, and how to get started.
What is Data Analytics?
Data analytics refers to the process of examining raw data to draw conclusions and insights. It involves the collection, cleaning, processing, and analyzing data to help organizations make informed decisions. The goal of data analytics is to identify patterns, trends, and relationships within data that can be used to solve problems or improve business performance.
Why is Data Analytics Important?
Data analytics is crucial for various reasons:
Informed Decision Making: Businesses use data to make more informed decisions based on facts and trends.
Improved Efficiency: By analyzing data, organizations can optimize their operations and reduce waste.
Predictive Insights: Data analytics can predict future trends, which help in strategic planning.
Competitive Advantage: Companies that leverage data analytics gain a competitive edge by responding quickly to market demands and customer needs.
Key Types of Data Analytics
Data analytics can be broken down into four main types:
1. Descriptive Analytics
Descriptive analytics focuses on summarizing historical data to understand what has happened. It provides a clear picture of past performance using reports, charts, and graphs.
Example: Analyzing sales data to see how many products were sold in the past quarter.
2. Diagnostic Analytics
Diagnostic analytics helps determine the cause of a particular trend or outcome. It answers the question: "Why did this happen?"
Example: If sales dropped in a particular region, diagnostic analytics can identify factors such as pricing changes, marketing efforts, or external factors like seasonality.
3. Predictive Analytics
Predictive analytics uses statistical models and machine learning to forecast future trends. It answers the question: "What is likely to happen next?"
Example: Using historical sales data to predict future demand for a product.
4. Prescriptive Analytics
Prescriptive analytics goes a step further and provides recommendations for actions. It answers the question: "What should we do about it?"
Example: A recommendation system that suggests products to users based on their browsing history.
Key Steps in the Data Analytics Process
The data analytics process typically follows these essential steps:
Step 1: Define Your Objective
Before starting any analysis, it’s crucial to define your objective. What problem are you trying to solve? What questions do you want to answer? Having clear goals will guide the entire analytics process.
Step 2: Collect Data
Data collection is the process of obtaining pertinent information from multiple sources.This could include internal data (e.g., sales reports, customer surveys) or external data (e.g., social media, public datasets).
Step 3: Clean the Data
Data cleaning is a vital step, as raw data is often incomplete, inconsistent, or noisy. Cleaning involves handling missing values, correcting errors, and transforming the data into a format that is easier to analyze.
Step 4: Analyze the Data
After the data is prepared, you can begin analyzing it. This may involve running statistical tests, building models, or using data visualization techniques to identify patterns and trends.
Step 5: Interpret the Results
The analysis must be interpreted in the context of the defined objectives. This is where the insights drawn from the data can inform decision-making or recommendations.
Step 6: Communicate Findings
Effective communication of findings is crucial for stakeholders to understand the implications of the analysis. Presenting results using visualizations such as charts and graphs can make complex data easier to comprehend.
Essential Data Analytics Tools for Beginners
As a beginner, using the right tools is crucial to streamline your data analytics workflow. Here are a few user-friendly tools for beginners:
1. Microsoft Excel
Microsoft Excel is one of the most widely used tools for data analysis. It allows users to create basic reports, perform statistical analysis, and visualize data through graphs and charts. It is an excellent starting point for beginners.
2. Google Analytics
Google Analytics is a powerful tool for analyzing web traffic and user behavior. It provides insights into website performance, conversion rates, and user demographics. This tool is especially useful for those interested in digital marketing analytics.
3. Python and R
Python and R are widely used programming languages for data analysis.Both offer libraries like Pandas, NumPy, and Matplotlib (in Python) and ggplot2 (in R) for data manipulation, analysis, and visualization. These languages are ideal for more advanced analytics tasks.
4. Tableau
Tableau is a data visualization tool that helps turn raw data into interactive and shareable dashboards. It’s beginner-friendly but also has advanced features that cater to seasoned data analysts.
5. Power BI
Power BI is another great tool for data visualization and business intelligence. It integrates with Microsoft Office products and offers an easy interface to create dynamic dashboards and reports.
Skills Needed for Data Analytics
To become proficient in data analytics, it’s essential to develop certain skills:
1. Statistical Knowledge
Understanding statistics is crucial for data analysis. Basic concepts like mean, median, standard deviation, correlation, and regression will help you interpret data and draw accurate conclusions.
2. Programming Skills
While not always mandatory, programming skills can make data analysis more efficient. Learning Python or R will allow you to automate tasks, manipulate large datasets, and build more advanced models.
3. Data Visualization
The ability to present data in a meaningful way is important. Visualization tools such as charts, graphs, and dashboards can help you communicate your findings to stakeholders effectively.
4. Data Cleaning and Transformation
Data is often messy. Knowing how to clean and transform it into a usable format is an important skill for any data analyst.
5. Business Acumen
Understanding the business context is critical. Data analytics is not just about working with numbers but using those numbers to solve real-world business problems.
Getting Started with Data Analytics in 2025
Here’s how you can begin your data analytics journey:
1. Learn the Basics
Start by learning the fundamentals of data analytics. Online platforms like Coursera, edX, and Udemy offer beginner-friendly courses in data analysis, Python, and R.
2. Practice with Real Data
Start practicing with real-world datasets. Websites like Kaggle, Data.gov, and UCI Machine Learning Repository provide free datasets to work with.
3. Master Key Tools
Learn how to use tools like Excel, Python, or Tableau to analyze and visualize data. Start small and gradually increase the complexity of your projects.
4. Build a Portfolio
As you gain more skills, build a portfolio of projects to showcase your work. This will help you demonstrate your capabilities to potential employers.
5. Stay Updated
The field of data analytics is always evolving. Stay updated with the latest trends, tools, and techniques by following blogs, attending webinars, and joining online communities.
Conclusion
Data analytics is an essential skill in 2025 that can transform how businesses make decisions, optimize operations, and understand their customers. As a beginner, it’s important to start with the basics, build a strong foundation, and practice consistently. With the right tools, skills, and mindset, you can master data analytics and unlock exciting opportunities in this growing field. Enrolling in a Best Data Analytics course provider in Delhi, Noida, Mumbai, Indore, and other parts of India can be a great way to get started and gain hands-on experience with industry-relevant tools and techniques.
Comments