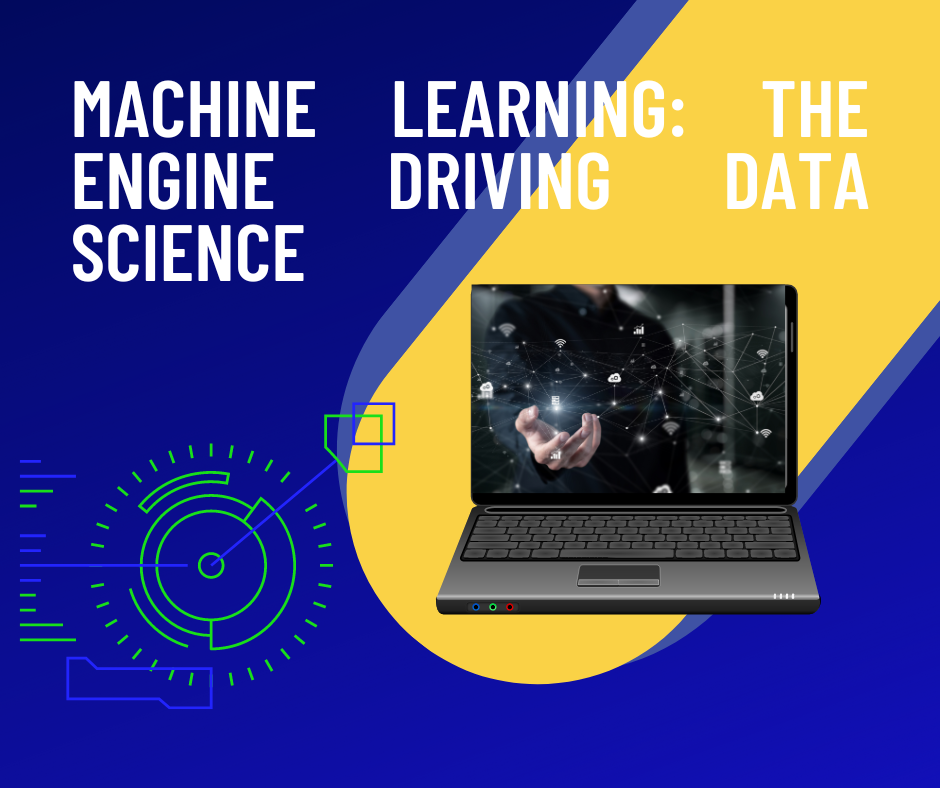
In today's data-rich world, businesses and organizations increasingly rely on data science to extract valuable insights and make informed decisions. At the heart of data science lies machine learning, a powerful tool that enables computers to learn from data without explicit programming. In this article, we'll explore the role of machine learning in driving data science forward, its applications across various industries, and its future potential.
Understanding Machine Learning
Machine learning is about building algorithms that learn from data to make predictions or decisions. These algorithms improve their performance over time without explicit programming. Machine learning can be broadly categorized into three types: supervised learning, unsupervised learning, and reinforcement learning.
Supervised Learning:
Supervised learning involves training a model on labeled data, where the model learns to map inputs to outputs based on example input-output pairs. For example, in a spam detection system, the model is trained on labeled emails (spam or not spam) to learn the patterns that distinguish between them.
Unsupervised Learning:
Unsupervised learning involves training a model on unlabeled data to find patterns or structures within the data. For example, clustering algorithms can be used to group similar data points together without prior knowledge of the groups.
Reinforcement Learning:
Reinforcement learning trains agents to make sequential decisions by interacting with an environment. The agent learns to take actions that maximize a reward signal, leading to a desired outcome. This approach is often used in game playing and robotics.
Applications of Machine Learning in Data Science
Machine learning has wide-ranging applications in data science across industries such as healthcare, finance, and marketing.
Healthcare:
In healthcare, machine learning is used to analyze medical images, predict patient outcomes, and personalize treatment plans. For example, machine learning models can help identify patterns in medical images to aid in the early detection of diseases like cancer.
Finance:
In finance, machine learning is used for fraud detection, algorithmic trading, credit scoring, and risk management. Machine learning algorithms can analyze vast amounts of financial data to detect unusual patterns that may indicate fraudulent activity.
Marketing:
In marketing, machine learning is used for customer segmentation, personalized recommendations, and targeted advertising. Machine learning models can analyze customer behavior and preferences to tailor marketing campaigns for maximum effectiveness.
Future Potential of Machine Learning in Data Science
As data continues to grow in volume and complexity, the role of machine learning in data science is expected to become even more critical. Advancements in machine learning techniques, such as deep learning, are enabling more complex and accurate models to be built.
Deep Learning:
Deep learning focuses on building neural networks with multiple layers. These deep neural networks have shown remarkable performance in tasks such as image recognition, natural language processing, and speech recognition.
Challenges and Considerations
While machine learning offers tremendous potential, it also comes with challenges. One key challenge is the need for large amounts of high-quality data for training. Without sufficient data, machine learning models may not generalize well to new, unseen data.
Another challenge is the interpretability of machine learning models. Some complex models, such as deep neural networks, are often referred to as "black boxes" because it can be difficult to understand how they arrive at their decisions. This lack of transparency can be a concern, especially in applications where decisions have significant consequences, such as healthcare or finance.
Ethical and Legal Implications
The increasing use of machine learning in data science raises important ethical and legal implications. For example, there are concerns about bias in machine learning models, where the models may reflect or amplify existing societal biases present in the training data. Addressing these biases requires careful consideration and monitoring of the data and the model's performance.
Future Directions
Looking ahead, the future of machine learning in data science is likely to be shaped by advancements in areas such as explainable AI, federated learning, and ethical AI. Explainable AI aims to make machine learning models more transparent and understandable, enabling better trust and accountability.
Conclusion
In conclusion, machine learning is a powerful tool driving the field of data science forward. Its wide-ranging applications and ongoing advancements make it an essential component of modern data-driven decision-making. However, it's important to be aware of the challenges and considerations associated with machine learning, including the need for high-quality data, model interpretability, and ethical and legal implications. By addressing these challenges, we can harness the full potential of machine learning to drive innovation and create a positive impact across industries. When looking for the best Online Data Science training Course in Delhi, Noida, Lucknow, Mumbai, Bangalore, and other parts of India, it's essential to consider these factors to ensure a comprehensive and effective learning experience.
Comments