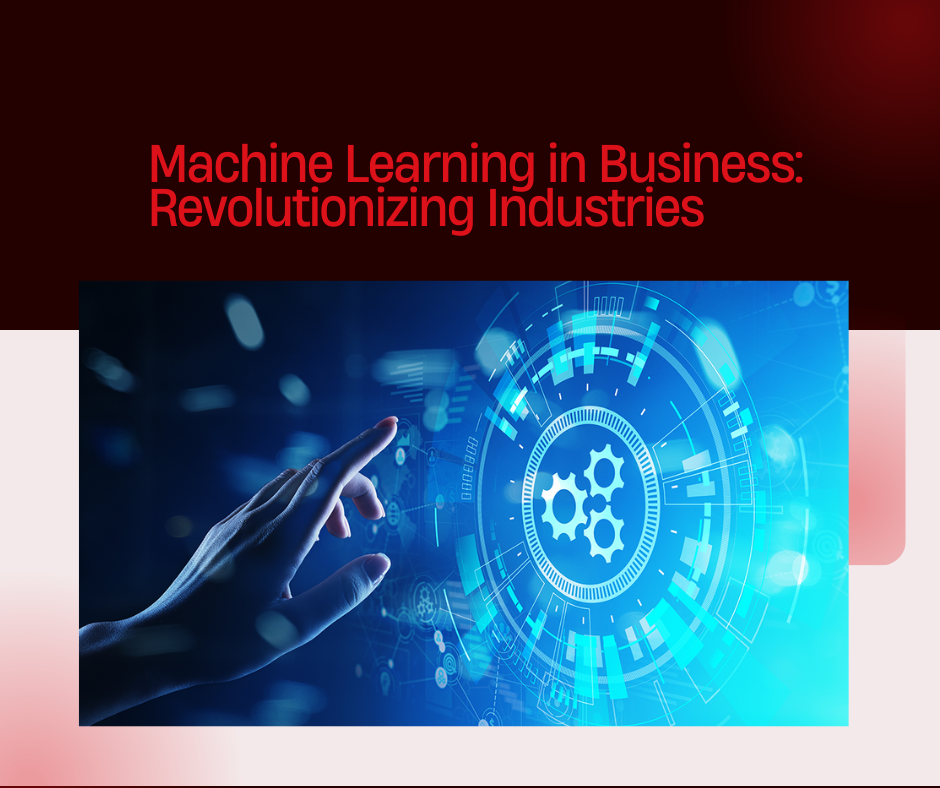
Machine learning (ML) is a subset of artificial intelligence (AI) that has transformed how businesses operate. With its ability to analyze large volumes of data, uncover patterns, and make predictions, machine learning is revolutionizing industries, driving innovation, and enabling businesses to remain competitive in an ever-evolving market.
In this article, we’ll explore how machine learning is being used in business, its benefits, applications, challenges, and the future of ML in business.
What is Machine Learning?
Machine learning refers to the use of algorithms and statistical models to enable computers to perform tasks without explicit programming. It allows systems to learn from data, improve their performance over time, and make decisions based on patterns and insights.
In a business context, ML can process and analyze vast amounts of data quickly, offering predictions, recommendations, and automated processes that support smarter decision-making.
How Does Machine Learning Work in Business?
Machine learning in business typically involves three main stages:
Data Collection: Gathering relevant data from various sources, such as customer interactions, sales records, or external market trends.
Prediction/Decision Making: The trained model makes predictions or informs decisions, improving over time as more data becomes available.
Machine learning models can be categorized into several types, such as supervised learning, unsupervised learning, and reinforcement learning, depending on the task they are designed to perform.
Benefits of Machine Learning for Businesses
1. Improved Decision-Making
ML empowers businesses to make data-driven decisions by identifying trends, predicting outcomes, and providing actionable insights. This leads to better strategic decisions that can enhance business performance.
2. Automation of Routine Tasks
Machine learning can automate repetitive and mundane tasks such as data entry, customer service responses, and inventory management. This allows employees to focus on higher-value tasks, improving productivity and reducing operational costs.
3. Personalized Customer Experiences
ML enables businesses to analyze customer data to understand preferences, behaviors, and needs. This information allows businesses to offer personalized recommendations, improve customer service, and increase customer satisfaction.
4. Fraud Detection
Machine learning is widely used in finance and banking to detect fraudulent activities. By analyzing transaction patterns and flagging anomalies, machine learning models can help identify potential fraud in real-time, protecting both businesses and customers.
5. Predictive Analytics
Predictive analytics powered by machine learning helps businesses forecast future trends, demand, and behaviors. For example, retailers can predict customer buying patterns and adjust inventory accordingly, while financial institutions can predict market trends.
6. Cost Reduction
ML algorithms help businesses optimize operations and reduce costs. By analyzing supply chains, production processes, and customer interactions, businesses can identify inefficiencies and implement cost-saving measures.
7. Enhanced Customer Support
Machine learning-powered chatbots and virtual assistants can handle customer queries, complaints, and service requests 24/7. These tools improve customer satisfaction while reducing the burden on human customer service teams.
Applications of Machine Learning in Business
1. Marketing and Advertising
Machine learning is transforming digital marketing by enabling businesses to target specific customer segments more effectively. By analyzing customer behavior, ML algorithms can predict which advertisements or content will resonate most with potential customers, leading to better conversion rates.
Key Applications:
Customer Segmentation: Grouping customers based on behavior, interests, and demographics.
Recommendation Engines: Personalizing product suggestions based on past behavior.
Ad Targeting: Optimizing ad placements and targeting based on user preferences.
2. Sales and Lead Generation
In sales, machine learning can predict which leads are most likely to convert into customers. ML algorithms analyze customer data, including interactions, past purchases, and demographic information, to score leads and prioritize them accordingly.
Key Applications:
Sales Forecasting: Predicting future sales based on historical data and trends.
Lead Scoring: Ranking potential leads based on their likelihood to convert.
Churn Prediction: Identifying customers who are likely to leave and providing insights for retention.
3. Customer Service
Machine learning is increasingly used in customer support to automate responses, classify tickets, and predict customer issues. AI-powered chatbots and virtual assistants can resolve simple queries, allowing human agents to handle more complex problems.
Key Applications:
Chatbots: Providing instant support through natural language processing (NLP).
Automated Ticket Routing: Assigning support tickets to the appropriate department based on content.
Sentiment Analysis: Analyzing customer feedback to gauge satisfaction and detect issues.
4. Supply Chain and Inventory Management
Machine learning helps businesses optimize supply chain processes by predicting demand, improving inventory management, and minimizing waste. It also aids in logistics by finding the most efficient routes and reducing shipping costs.
Key Applications:
Route Optimization: Finding the most cost-effective delivery routes.
Inventory Optimization: Minimizing overstock and stockouts to reduce inventory costs.
5. Human Resources
HR departments are increasingly turning to machine learning to enhance recruitment, employee retention, and talent management. ML models can help identify the best candidates by analyzing resumes, job descriptions, and interview feedback.
Key Applications:
Recruitment: Automating candidate screening and matching candidates with job requirements.
Employee Retention: Predicting which employees are at risk of leaving and suggesting interventions.
Performance Management: Analyzing employee performance data to identify strengths and areas for improvement.
6. Financial Analysis and Risk Management
In finance, machine learning models are used to predict market trends, assess risks, and optimize portfolios. ML helps financial institutions identify investment opportunities, detect fraud, and minimize risks.
Key Applications:
Algorithmic Trading: Using ML models to analyze market data and execute trades based on patterns.
Credit Scoring: Assessing the risk associated with lending to individuals or businesses.
Challenges of Implementing Machine Learning in Business
1. Data Quality and Availability
Machine learning models rely on large volumes of high-quality data to perform well. However, many businesses face challenges such as data silos, incomplete datasets, and low-quality data, which can significantly impact the effectiveness of their ML systems.
2. Complexity and Expertise
Machine learning is a complex field that requires specialized knowledge and expertise. Businesses may face challenges in finding skilled data scientists or ML engineers to develop and maintain ML models.
3. Integration with Existing Systems
Integrating machine learning models into existing business operations and systems can be difficult. It requires significant infrastructure changes, technical expertise, and ongoing maintenance.
4. Ethical Considerations
ML models are only as good as the data they are trained on. If the data is biased, the model's predictions may be flawed or discriminatory. Businesses must ensure they are using ethical practices when collecting and using data.
The Future of Machine Learning in Business
The future of machine learning in business looks promising, with new developments in AI, data analytics, and automation set to continue transforming industries. Key trends to watch for include:
Increased Automation: More businesses will rely on ML to automate complex tasks and processes, improving efficiency and reducing human error.
Enhanced Personalization: Businesses will leverage ML to offer highly personalized experiences, from marketing to customer service.
Edge Computing: Machine learning will be integrated with IoT devices, allowing real-time data processing and decision-making at the edge, rather than relying solely on centralized cloud computing.
AI Ethics and Regulation: As ML becomes more widespread, ethical considerations and regulatory frameworks will play an important role in guiding the development and use of AI technologies.
Conclusion
Machine learning is no longer a futuristic technology; it is here and is already making a significant impact across all industries. From improving decision-making and customer service to enhancing marketing and sales strategies, machine learning has become an essential tool for modern businesses. To stay ahead of the curve, professionals can enhance their expertise with Machine Learning Certification training in Noida, Delhi, Mumbai, Indore, and other parts of India, helping them harness the full potential of this transformative technology.
While challenges remain, the benefits far outweigh the risks, making machine learning a valuable asset for businesses looking to gain a competitive edge. As technology continues to evolve, the potential applications of ML in business will only expand, offering new opportunities for growth, efficiency, and innovation.
Comments