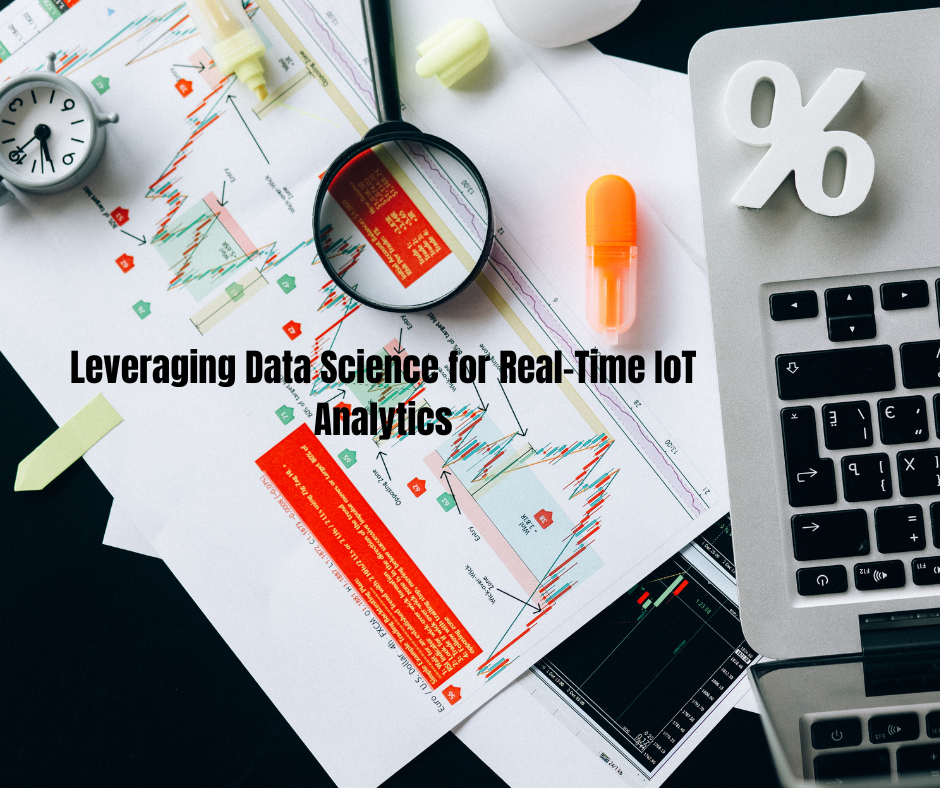
The Internet of Things (IoT) has transformed industries by enabling everyday devices to connect, communicate, and exchange data with one another and centralized systems. As the number of IoT devices grows exponentially, the data generated increases at a massive scale, making real-time analysis essential for deriving actionable insights. Data Science plays a crucial role in processing, analyzing, and extracting value from this data. By combining IoT with Data Science, organizations across sectors like healthcare, manufacturing, smart cities, and agriculture can unlock immense potential.
In this article, we’ll explore how Data Science is leveraged for real-time IoT analytics, the challenges it presents, and the opportunities it creates through this powerful combination.
What is Real-Time IoT Analytics?
Real-time IoT analytics refers to the continuous collection and analysis of data from IoT devices. The goal is to monitor, detect, and predict events as they occur, enabling fast decision-making and proactive responses. For instance, in a smart factory, IoT sensors track machinery performance, detect faults, and alert maintenance teams before a breakdown happens.
The Role of Data Science in IoT Analytics
Data Science provides structure and insight into the vast, unstructured data produced by IoT devices. It utilizes techniques like statistical analysis, machine learning, and predictive analytics to interpret raw data generated by sensors, devices, and systems.
Data Collection and Preprocessing: IoT devices continuously generate large amounts of data in various formats. This data often requires cleaning, normalization, and structuring before it’s ready for analysis. Data scientists use preprocessing techniques to filter out noise and irrelevant information, ensuring the data is prepared for meaningful analysis.
Real-Time Processing: Many IoT systems require real-time data processing, where information is analyzed as it’s generated. Traditional batch processing is insufficient for this purpose. Instead, stream processing frameworks like Apache Kafka, Apache Flink, or Spark Streaming handle real-time data streams, enabling immediate action when anomalies are detected or trends are identified.
Machine Learning for Predictive Analytics: Machine learning models are integral to IoT analytics, enabling predictive analytics to forecast future events or anomalies based on historical data. In predictive maintenance, for example, machine learning algorithms analyze equipment data to predict when machinery might fail, allowing for timely repairs.
Anomaly Detection: Anomaly detection is a key application of data science in IoT analytics. Anomalies, or deviations from the norm, may indicate malfunctions, security threats, or unusual behavior. Data scientists develop models that automatically detect and flag these anomalies in real-time, enabling rapid responses and mitigating potential risks.
Visualization and Insights: After processing and analyzing the data, it must be presented in a meaningful way. Data visualization tools like dashboards, graphs, and charts help monitor IoT systems. Real-time visualizations allow stakeholders to quickly gain insights and make data-driven decisions.
Use Cases of IoT and Data Science
The combination of IoT and Data Science is being applied across industries to improve efficiency, enhance safety, and optimize operations. Here are some key use cases:
Smart CitiesIn smart cities, IoT sensors monitor traffic, air quality, water usage, and energy consumption. Data science analyzes this data to inform decision-making. For instance, real-time traffic data can be used to optimize traffic light patterns, reducing congestion and improving urban mobility.Similarly, smart energy grids gather data from electricity meters and use machine learning models to predict energy demand, ensuring efficient energy distribution and minimizing waste.
HealthcareIn healthcare, IoT devices like wearables and smart medical devices monitor patients’ vital signs—heart rate, temperature, and blood pressure. Data science analyzes this information to detect anomalies, predict health risks, and trigger emergency responses when necessary.Predictive models, for example, can analyze heart rate data to identify patterns that may indicate an impending cardiac event, allowing healthcare providers to intervene early.
Manufacturing and Industrial IoT (IIoT)In industrial settings, IoT sensors monitor machinery, track inventory, and optimize production lines. Data science enables predictive maintenance, where machine learning models analyze sensor data to predict equipment failures before they happen. This reduces downtime and increases operational efficiency.Additionally, real-time analytics monitor supply chain operations, identify bottlenecks, and optimize processes to reduce costs and enhance productivity.
AgricultureSmart farming uses IoT devices to monitor soil conditions, weather patterns, and crop health. Data science analyzes this information to provide actionable insights, such as determining the best time for irrigation or fertilization.For example, machine learning models can predict crop yields based on historical weather data and soil conditions, helping farmers maximize productivity and reduce resource usage.
RetailRetailers use IoT devices like smart shelves, RFID tags, and customer tracking systems to monitor inventory, customer behavior, and sales trends. Data science helps analyze this data to understand customer preferences, optimize stock levels, and personalize shopping experiences.Real-time analytics can also trigger restocking when inventory falls below a threshold, ensuring that popular products are always available.
Challenges in Real-Time IoT Analytics
While the integration of Data Science and IoT presents significant opportunities, it also comes with challenges:
Data Volume and Velocity: IoT devices generate vast amounts of data at high speeds. Managing and processing this data in real-time requires robust infrastructure and scalable processing frameworks.
Data Quality and Noise: IoT data often contains noise, errors, or inconsistencies. Preprocessing is essential to clean and prepare the data for analysis, but it can be resource-intensive.
Security and Privacy: IoT devices are vulnerable to security threats like hacking and data breaches. Furthermore, the data they generate may include sensitive information, raising privacy concerns. Organizations must implement robust security measures and comply with privacy regulations.
Interoperability: IoT devices from different manufacturers may use varying protocols and standards, making it challenging to integrate and analyze data from diverse sources. Developing standardized frameworks for data exchange is crucial for seamless integration.
Scalability: As IoT ecosystems grow, the number of connected devices and the volume of data they produce will increase. Ensuring that analytics platforms can scale accordingly is critical for maintaining real-time analytics capabilities.
Future Trends in IoT Analytics
Several emerging trends will shape the future of real-time IoT analytics:
Edge Computing: Edge computing processes data closer to the source, reducing latency and bandwidth usage. As IoT ecosystems grow, edge computing will become vital for enabling real-time analytics where cloud computing might introduce delays.
AI-Powered Automation: AI will take on a larger role in automating IoT systems. AI algorithms will detect anomalies, make predictions, and trigger automated responses without human intervention, further improving the efficiency of IoT systems.
5G and Enhanced Connectivity: The rollout of 5G networks will improve IoT device connectivity, enabling faster data transmission and supporting a higher density of connected devices. This will allow for more advanced real-time analytics, supporting use cases like autonomous vehicles and smart cities.
Conclusion
Leveraging Data Science for real-time IoT analytics offers transformative potential across industries. By combining IoT’s vast data generation with Data Science’s advanced analytical techniques, organizations can gain real-time insights, enhance decision-making, and optimize operations. For those seeking to harness this potential, pursuing the Best Data Science Training in Delhi, Noida, Mumbai, Indore, and other parts of India can provide the necessary skills and knowledge. However, challenges like data volume, security, and scalability must be addressed to fully unlock this potential. As IoT ecosystems continue to evolve, and with the emergence of technologies like AI and edge computing, real-time IoT analytics will become even more powerful, shaping the future of industries globally.
Comentários