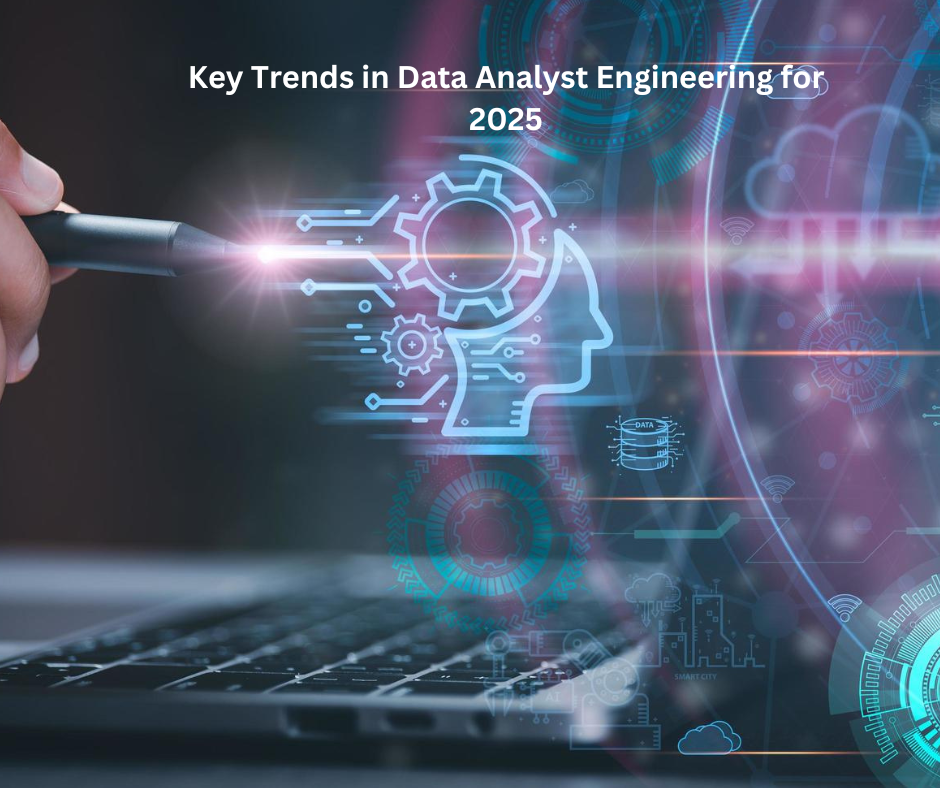
Data analyst engineering continues to evolve as organizations increasingly rely on data-driven insights. In 2025, this field is expected to experience significant advancements, driven by technological innovations, changing business needs, and the growing importance of data governance. Below, we explore the key trends shaping data analyst engineering in 2025.
1. Adoption of Artificial Intelligence and Machine Learning
Artificial Intelligence (AI) and Machine Learning (ML) are becoming integral to data analyst engineering. By 2025, these technologies will enable:
Automated Data Analysis: Tools that use AI to uncover patterns, correlations, and trends in massive datasets with minimal human intervention.
Predictive Analytics: Machine learning algorithms that forecast future trends and behaviors based on historical data.
Anomaly Detection: Real-time identification of outliers, fraud, and operational issues.
As AI and ML integrate into data pipelines, data analysts will focus more on interpreting insights and less on manual data manipulation.
2. Emphasis on Real-Time Data Analytics
The demand for real-time insights is driving a shift towards streaming data analytics. In 2025, organizations will prioritize:
Faster Decision-Making: Leveraging tools like Apache Kafka and Spark Streaming to process live data feeds.
IoT Integration: Managing data from Internet of Things (IoT) devices to monitor operations in industries like manufacturing, healthcare, and logistics.
Dynamic Dashboards: Visualizations that update instantly, allowing decision-makers to act on current information.
Real-time analytics will be crucial for competitive industries, ensuring rapid responses to market changes.
3. Increased Focus on Data Privacy and Security
With stricter regulations like GDPR, CCPA, and others, data privacy and security are top priorities in 2025. Key developments include:
Advanced Encryption Techniques: Protecting sensitive data during storage and transmission.
Data Masking and Tokenization: Concealing identifiable information to reduce risk while maintaining data usability.
Compliance Automation: Tools that ensure adherence to global data protection laws.
Data analysts must align with legal and ethical standards, ensuring data handling practices remain compliant.
4. Rise of Data Mesh Architecture
Traditional centralized data platforms are giving way to decentralized approaches like data mesh. By 2025, this architecture will:
Empower Teams: Grant domain teams ownership of their data pipelines, improving agility.
Enhance Scalability: Reduce bottlenecks by decentralizing responsibilities across multiple teams.
Improve Data Accessibility: Foster collaboration and innovation by democratizing data.
Data mesh will transform how organizations design, manage, and utilize their data infrastructure.
5. Self-Service Business Intelligence (BI)
Self-service BI tools enable non-technical users to analyze and visualize data independently. In 2025, these tools will:
Simplify Data Access: Provide user-friendly interfaces for querying and reporting.
Encourage Collaboration: Facilitate cross-departmental data sharing and decision-making.
Reduce Dependence on IT: Minimize the backlog of requests for data insights.
Popular tools like Tableau, Power BI, and Looker will become more intuitive, making data analysis accessible to all.
6. Cloud-Native Data Solutions
Cloud computing continues to dominate data analytics. By 2025, cloud-native solutions will:
Support Scalability: Adapt to fluctuating data volumes and processing needs.
Enhance Collaboration: Allow teams to access data and tools from anywhere in the world.
Reduce Costs: Eliminate the need for extensive on-premise infrastructure.
Cloud providers like AWS, Azure, and Google Cloud will introduce more sophisticated tools tailored to data analytics.
7. Advanced Data Visualization Techniques
Data visualization is evolving beyond static charts and graphs. By 2025, advancements will include:
Interactive Visualizations: Dashboards that allow users to drill down into data layers.
Augmented Reality (AR) and Virtual Reality (VR): Immersive tools for exploring complex datasets.
Storytelling with Data: Narratives that make insights more engaging and understandable.
These techniques will enhance the ability of data analysts to communicate findings effectively.
8. Growth of Low-Code and No-Code Platforms
Low-code and no-code platforms are reducing barriers to entry in data analytics. In 2025, these platforms will:
Enable Rapid Development: Build data workflows and dashboards without extensive programming knowledge.
Empower Non-Technical Users: Allow business stakeholders to create their own reports and models.
Increase Productivity: Free up data engineers and analysts for more complex tasks.
As these platforms become more robust, organizations will embrace them for faster implementation.
9. Integration of Edge Computing
Edge computing processes data closer to its source, such as IoT devices or local servers. By 2025, its role in data analytics will include:
Reduced Latency: Processing data locally for faster insights.
Enhanced Security: Minimizing the risk of data breaches by keeping data on-site.
Support for Remote Locations: Enabling analytics in areas with limited internet connectivity.
Edge computing will complement cloud solutions, especially for time-sensitive applications.
10. Data Democratization
In 2025, organizations will continue to prioritize data democratization, ensuring data is accessible to all employees. This trend will involve:
Data Literacy Training: Equipping employees with the skills to interpret and utilize data effectively.
Centralized Data Catalogs: Providing a single source of truth for all organizational data.
Transparent Governance: Defining clear policies for data access and usage.
Data democratization fosters a culture of informed decision-making across all levels of an organization.
11. Evolution of Data Analyst Roles
As data analyst engineering evolves, so do the roles and responsibilities within the field. By 2025, we’ll see:
Hybrid Roles: Combining data analysis with skills in engineering, visualization, or domain expertise.
Specialization: Roles like data storyteller, data product manager, and AI ethicist emerging.
Focus on Soft Skills: Emphasizing communication, collaboration, and critical thinking.
These changes will create more dynamic and rewarding career opportunities in data analytics.
12. Ethical AI and Responsible Data Use
With the rise of AI, ethical concerns around bias, fairness, and transparency are growing. In 2025, organizations will:
Audit AI Models: Ensure fairness and eliminate unintended biases.
Adopt Transparent Practices: Clearly explain how data is collected, processed, and used.
Promote Inclusivity: Design solutions that consider diverse user needs.
Ethical AI practices will be integral to building trust and sustainability in data-driven businesses.
Conclusion
The field of data analyst engineering is undergoing rapid transformation, driven by advancements in technology, changing market demands, and the need for responsible data management. Trends like AI integration, real-time analytics, data democratization, and ethical AI will redefine how data analysts work and the value they deliver to organizations. To stay ahead in this evolving field, professionals can benefit from the Best Data Analytics Training in Greater Noida, Delhi, Noida, Mumbai, Indore, and other parts of India, which equips them with the latest skills and knowledge to excel in the industry.
Comments