How Data Science is Transforming Healthcare
- Ruhi Parveen
- Sep 6, 2024
- 6 min read
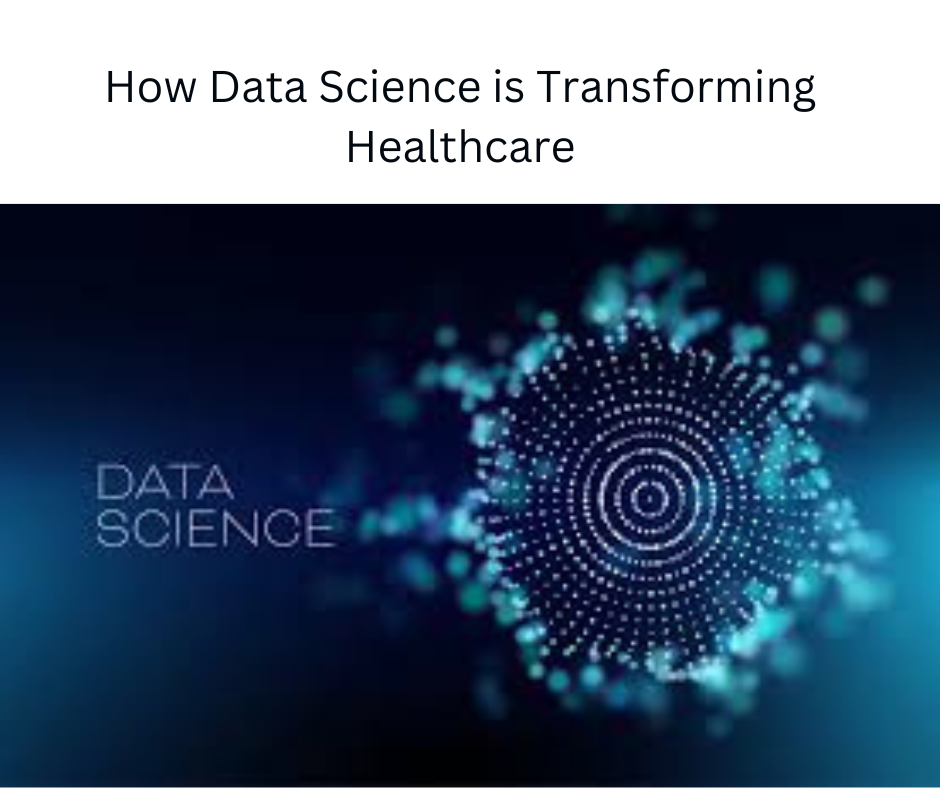
Data science is revolutionizing the healthcare industry by enabling more efficient, accurate, and personalized care. The application of big data, machine learning, and artificial intelligence (AI) in healthcare is improving diagnostics, treatment, and patient management. From predictive analytics to personalized medicine, data science is reshaping healthcare in many ways. Let's explore how this transformation is happening.
1. Introduction to Data Science in Healthcare
Data science involves the use of algorithms, statistical methods, and machine learning models to extract insights from large datasets. In healthcare, data science is used to analyze patient records, clinical trials, and other medical data to predict outcomes, enhance treatments, and reduce healthcare costs.
With the rise of electronic health records (EHRs) and advanced medical imaging technologies, healthcare organizations now have access to vast amounts of data. This data can be leveraged to improve patient outcomes, reduce errors, and optimize healthcare delivery.
2. The Role of Big Data in Healthcare
Big data in healthcare refers to the collection of massive amounts of patient information, such as medical histories, treatment records, and diagnostic images. These datasets can be difficult to process with traditional methods, but data science techniques allow for the analysis of these large and complex datasets.
Key Benefits of Big Data in Healthcare:
Predictive Analytics: By analyzing patient data, healthcare professionals can predict disease outbreaks, patient readmission risks, and potential treatment responses.
Improved Diagnostics: Big data enables faster and more accurate diagnosis through the analysis of imaging data, laboratory results, and genetic information.
Operational Efficiency: Hospitals and clinics can optimize staffing, reduce wait times, and enhance the overall patient experience by analyzing administrative data.
3. Predictive Analytics for Disease Prevention
One of the most impactful uses of data science in healthcare is predictive analytics. Predictive analytics involves using data from past patients to make predictions about future health outcomes. It allows healthcare providers to anticipate diseases before they become critical.
Example of Predictive Analytics:
Heart Disease Prediction: By analyzing data such as patient lifestyle, genetic history, and clinical records, data science algorithms can predict the likelihood of heart disease. This helps doctors initiate early interventions, reducing the risk of fatal outcomes.
Cancer Screening: Predictive analytics can identify patterns that suggest cancer risks, allowing for earlier detection and treatment.
4. Personalized Medicine and Precision Healthcare
Data science is enabling a shift from a "one-size-fits-all" approach to more personalized healthcare. Personalized medicine uses patient data, such as genetic information and lifestyle factors, to tailor treatments to individual needs.
Benefits of Personalized Medicine:
Tailored Treatments: Data science allows doctors to prescribe medications that are more likely to be effective for a specific patient based on their genetic makeup and medical history.
Fewer Side Effects: By analyzing patient data, doctors can identify which treatments are least likely to cause side effects, improving patient comfort and outcomes.
Genomic Medicine: Genomic data can be analyzed to understand how different people respond to treatments, leading to more effective therapies for diseases like cancer.
5. Drug Discovery and Development
The drug discovery process is traditionally time-consuming and expensive, but data science is speeding up the process by enabling researchers to analyze vast amounts of biological data more efficiently. AI algorithms can predict how different molecules will interact with diseases, allowing researchers to identify potential new drugs more quickly.
Key Contributions of Data Science in Drug Development:
Accelerated Research: Machine learning models can analyze millions of compounds in a fraction of the time it would take using traditional methods.
Predicting Drug Efficacy: Data science models can predict how well a drug will work for different patient populations, reducing the time and cost of clinical trials.
Repurposing Existing Drugs: Data science can also identify new uses for existing drugs, accelerating the availability of treatments for diseases that currently lack effective therapies.
6. AI and Machine Learning in Medical Imaging
Medical imaging is a critical part of diagnostics, and data science is transforming how images are analyzed. AI and machine learning algorithms can analyze medical images such as X-rays, MRIs, and CT scans faster and with greater accuracy than human radiologists.
AI Applications in Medical Imaging:
Early Disease Detection: AI algorithms can identify patterns in medical images that may indicate diseases such as cancer or brain disorders at early stages, even before symptoms appear.
Reduction in Human Error: Machine learning models are not prone to fatigue, making them less likely to miss critical details in medical images.
Faster Diagnosis: With AI assistance, doctors can analyze scans more quickly, speeding up the diagnostic process and allowing for faster treatment.
7. Enhancing Patient Care with Wearable Devices
Wearable technology, such as fitness trackers and smartwatches, is providing real-time health data on heart rate, activity levels, and sleep patterns. Data science tools can process this data to monitor patient health remotely and in real time.
Benefits of Wearable Devices in Healthcare:
Chronic Disease Management: Wearables can help monitor chronic conditions such as diabetes or hypertension, alerting patients and doctors when intervention is needed.
Preventive Care: These devices can detect irregular heartbeats or other early signs of health issues, prompting users to seek medical attention before a problem becomes severe.
Data for Clinical Decisions: Doctors can use data from wearables to track patient health trends and adjust treatment plans accordingly.
8. Healthcare Operations and Resource Management
Data science is not only transforming patient care but also enhancing operational efficiency in healthcare facilities. By analyzing administrative and patient flow data, hospitals can optimize their resources, reducing costs and improving service delivery.
Applications of Data Science in Healthcare Operations:
Staff Scheduling: Data analysis helps predict peak times and patient volumes, allowing hospitals to allocate staff more effectively.
Supply Chain Optimization: Data science can optimize the supply chain for medical supplies and pharmaceuticals, ensuring that resources are available when needed.
Patient Flow Management: Hospitals can analyze data to predict patient admission rates and optimize bed management, reducing wait times and improving patient satisfaction.
9. Telemedicine and Remote Care
The COVID-19 pandemic accelerated the adoption of telemedicine, and data science is playing a crucial role in its continued development. Telemedicine platforms can collect and analyze patient data, enabling healthcare providers to make informed decisions during remote consultations.
How Data Science Supports Telemedicine:
Remote Monitoring: Data collected from patients through telemedicine platforms, such as vital signs and symptoms, can be analyzed to detect early warning signs of deterioration.
Personalized Virtual Care: Data science enables more personalized remote consultations by analyzing patient histories and offering tailored treatment recommendations.
Improved Access to Care: Telemedicine, supported by data science, ensures that patients in remote or underserved areas have access to quality healthcare.
10. Ethical Considerations and Challenges
Despite the numerous benefits, the use of data science in healthcare also presents several challenges, especially in terms of privacy and ethics.
Key Ethical Concerns:
Data Privacy: With the collection of vast amounts of sensitive health information, protecting patient data from breaches is a top priority.
Bias in Algorithms: Data science models can inherit biases from the data they are trained on, leading to unequal treatment of different patient groups.
Data Security: As healthcare becomes more digitized, ensuring the security of patient data against cyberattacks is critical.
11. Future of Data Science in Healthcare
The future of healthcare lies in leveraging data science to deliver more accurate, personalized, and cost-effective care. As AI and machine learning technologies continue to advance, we can expect further innovations in areas such as diagnostics, treatment planning, and patient management.
Emerging Trends:
AI-Powered Assistants: Virtual assistants will become more common in healthcare, helping doctors analyze data and make more informed decisions.
Real-Time Analytics: The ability to analyze patient data in real time will revolutionize emergency care and chronic disease management.
Integration of Genomics: Genomic data analysis will allow for even more personalized treatments based on an individual's genetic makeup.
12. Conclusion
Data science is transforming every aspect of healthcare, from patient diagnosis and treatment to hospital operations and drug development. The ability to analyze vast amounts of data is enabling healthcare professionals to make better decisions, improve patient outcomes, and reduce costs. To harness this potential effectively, healthcare professionals can benefit from the Best Data Science Training in Noida,Delhi , Mumbai, Indore, and other parts of India, which equips them with the necessary skills to leverage data for improved healthcare outcomes. However, it is essential to address ethical concerns to ensure that the benefits of data science are realized in a fair and secure manner. As technology continues to advance, the potential for data science to further revolutionize healthcare is limitless.
Comments