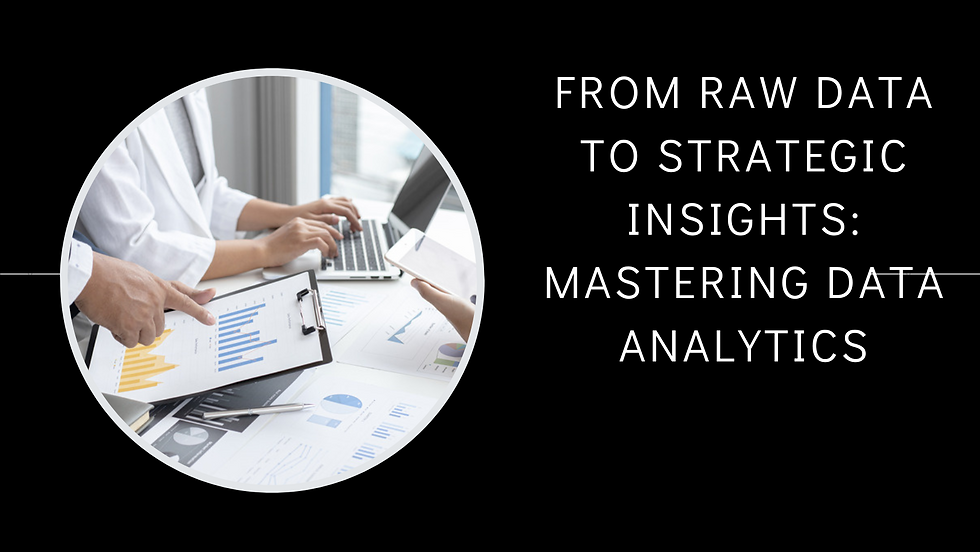
In today's data-driven landscape, the journey from raw data to strategic insights is a critical aspect of organizational success. This article delves into the realm of data analytics, exploring its fundamental principles, methodologies, and the transformative impact it has on decision-making processes.
Understanding Data Analytics
What is Data Analytics?
Data analytics involves the systematic analysis of raw data to uncover patterns, trends, correlations, and meaningful insights. It utilizes various techniques and tools to convert vast amounts of information into actionable knowledge, aiding businesses in making informed decisions.
Components of Data Analytics:
Data Collection:
The process begins with the gathering of raw data from diverse sources such as databases, spreadsheets, and external APIs.
Data Cleaning:
Preprocessing involves cleaning the data to handle missing values, errors, and inconsistencies, ensuring its accuracy and reliability.
Exploratory Data Analysis (EDA):
EDA involves visualizing and summarizing data to understand its distribution, patterns, and outliers, guiding subsequent analyses.
Statistical Analysis:
Leveraging statistical methods to derive meaningful insights and draw inferences from the data.
Data Modeling:
Constructing models to represent relationships within the data and predict future outcomes.
Machine Learning:
Implementing machine learning algorithms to automate analytical model building and improve decision-making processes.
Data Visualization:
Presenting complex data in visual formats, such as charts and graphs, to facilitate understanding and communication.
The Data Analytics Process: A Step-by-Step Guide
1. Define Objectives:
Clearly define the objectives of the analysis, ensuring alignment with organizational goals.
2. Data Collection:
Gather relevant data from internal and external sources, ensuring its quality and relevance to the analysis.
3. Data Cleaning:
Cleanse the data to address missing values, errors, and inconsistencies, enhancing its reliability.
4. Exploratory Data Analysis (EDA):
Visualize and summarize the data to identify patterns, trends, and outliers, informing subsequent analysis.
5. Statistical Analysis:
Apply statistical techniques to derive insights and make data-driven decisions.
6. Data Modeling:
Construct models to represent relationships within the data and make predictions.
7. Machine Learning:
Implement machine learning algorithms to automate and enhance analytical processes.
8. Data Visualization:
Present the results in visual formats, making complex information accessible and understandable.
9. Interpretation:
Interpret the findings in the context of the defined objectives, drawing actionable insights.
10. Communication:
Effectively communicate the results and insights to stakeholders, facilitating informed decision-making.
Applications of Data Analytics
1. Business Intelligence:
Data analytics drives business intelligence by providing insights into market trends, customer behavior, and competitive landscapes.
2. Marketing Optimization:
Analyzing customer data enables targeted marketing campaigns, personalized recommendations, and improved customer engagement.
3. Financial Analysis:
In finance, data analytics aids in risk management, fraud detection, and portfolio optimization.
4. Healthcare:
Patient data analysis enhances medical research, diagnosis, and treatment planning.
5. Operations and Supply Chain Management:
Optimizing processes and predicting demand through data analytics improves efficiency in operations and supply chain management.
6. Human Resources:
Analyzing employee data supports talent acquisition, performance management, and workforce planning.
7. Predictive Maintenance:
In industries such as manufacturing, data analytics is used for predicting equipment failures and optimizing maintenance schedules.
Key Technologies in Data Analytics
1. Data Warehousing:
Centralized storage and retrieval of large volumes of structured and unstructured data.
2. Data Mining:
Extracting patterns and knowledge from large datasets using statistical methods.
3. Predictive Analytics:
Using statistical algorithms and machine learning techniques to identify future trends and outcomes.
4. Business Intelligence Tools:
Platforms like Tableau, Power BI, and Qlik for visualizing and interpreting data.
5. Natural Language Processing (NLP):
Enabling machines to understand, interpret, and generate human-like text.
6. Cloud Computing:
Providing scalable and cost-effective infrastructure for data storage and processing.
Challenges in Data Analytics
1. Data Quality:
Poor data quality can lead to inaccurate analyses and unreliable insights.
2. Privacy Concerns:
Handling sensitive information raises ethical and legal considerations.
3. Skill Gap:
The demand for skilled data analysts often surpasses the available talent pool.
4. Integration Issues:
Integrating data analytics into existing business processes can be challenging.
5. Rapid Technological Advancements:
Staying updated with the latest tools and technologies is essential in this dynamic field.
The Future of Data Analytics
The future of data analytics holds promising developments:
1. Artificial Intelligence Integration:
AI will play a more significant role, automating complex analytical tasks and uncovering deeper insights.
2. Edge Analytics:
Processing data closer to its source for real-time analytics, reducing latency.
3. Augmented Analytics:
Combining machine learning and AI to enhance data analytics capabilities, making insights more accessible to a broader audience.
4. Continued Focus on Ethics:
Ethical considerations surrounding data usage and privacy will remain a focal point.
5. Democratization of Data:
Increasing accessibility to data analytics tools, empowering individuals across various roles to leverage data for decision-making.
Conclusion
For those aspiring to gain expertise in data analytics, finding the right training institute is crucial. A reputable Data Analytics Training Institute in Lucknow, Delhi, Noida, Indore, Bangalore and all cities in India can provide the necessary knowledge and skills. These institutes offer comprehensive courses covering data collection, cleaning, exploratory data analysis, statistical analysis, modeling, machine learning, and data visualization. Aspiring professionals can benefit from hands-on experience and guidance from industry experts, preparing them to contribute effectively to the growing field of data analytics. Choosing the right training institute is a key step in empowering individuals and organizations to leverage the full potential of data for strategic decision-making.
Comentarios