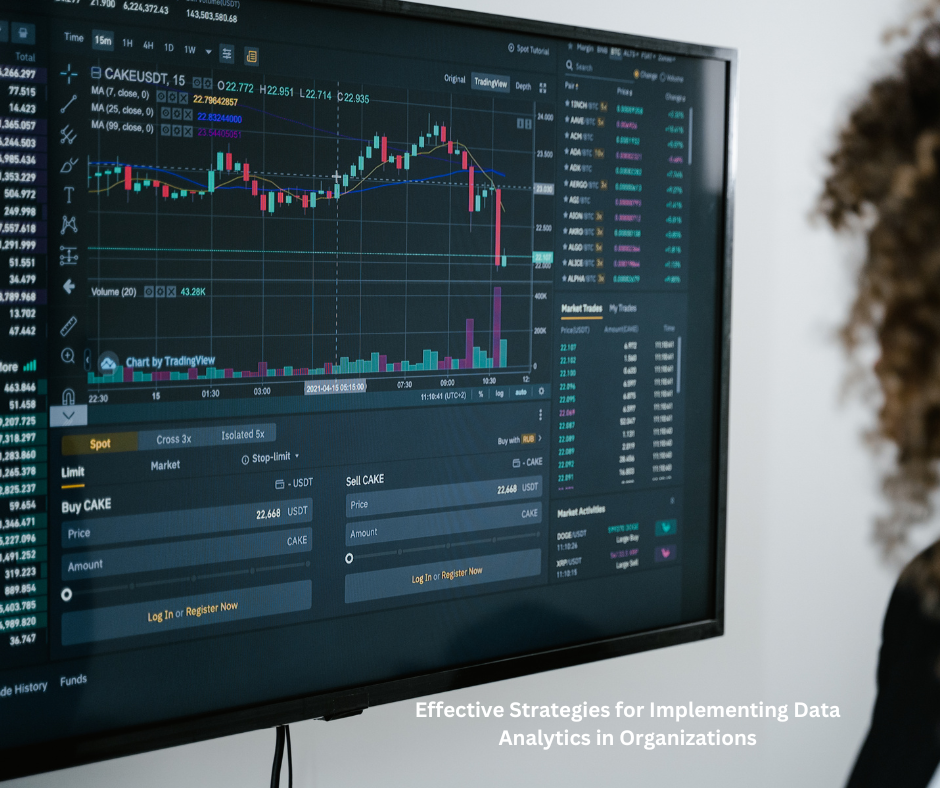
Data analytics has become an essential component of modern business operations. It enables organizations to derive valuable insights from vast amounts of data, leading to better decision-making, enhanced operational efficiency, and improved customer experiences. However, implementing data analytics effectively can be a challenging task. In this guide, we will explore the most effective strategies for successfully integrating data analytics into an organization.
Understand the Importance of Data Analytics
Before embarking on the implementation process, it's essential to grasp the importance of data analytics.Organizations that harness the power of data analytics can:
Make informed decisions: Data-driven decision-making eliminates guesswork and supports strategic choices backed by concrete insights.
Optimize operations: Analytics can streamline processes, reduce inefficiencies, and improve overall business performance.
Enhance customer experience: By analyzing customer behavior, preferences, and feedback, companies can deliver personalized services and improve satisfaction.
Drive innovation: With data insights, businesses can identify emerging trends, gaps in the market, and new opportunities for growth.
Define Clear Objectives
One of the first steps in implementing data analytics is defining the specific goals and objectives for your organization. These objectives will guide the entire process and ensure that efforts are focused and aligned with business priorities.
Key Questions to Consider:
What business problems do we want to solve with data analytics?
Are we aiming to improve customer retention, boost sales, optimize supply chains, or enhance operational efficiency?
How will data analytics contribute to our overall business strategy?
By answering these questions, you can ensure that the data analytics initiatives are aligned with the organization’s vision and mission.
Invest in the Right Tools and Technology
Choosing the right data analytics tools and technologies is fundamental to the success of your implementation efforts. The market is full of a wide range of analytics platforms, from basic data visualization tools to sophisticated artificial intelligence and machine learning platforms. To make a well-informed decision, take the following factors into account:
a) Data Integration
Ensure that the chosen tools can integrate with your existing systems. You may need tools that can connect with customer relationship management (CRM) systems, enterprise resource planning (ERP) platforms, or supply chain management software.
b) Scalability
Select platforms that can scale as your data grows. The tool should handle increasing volumes of data and more complex analyses as the organization expands.
c) Ease of Use
The tools should be user-friendly and accessible to both data analysts and non-technical users. This encourages wider adoption across departments and improves the efficiency of decision-making.
d) Advanced Analytics Capabilities
Consider tools that offer advanced analytics capabilities, such as predictive analytics, machine learning, and natural language processing, depending on your objectives.
Build a Data-Driven Culture
To fully leverage data analytics, it’s crucial to foster a data-driven culture within the organization. This involves embedding data-driven decision-making into the daily processes and mindset of employees at all levels.
Steps to Build a Data-Driven Culture:
Promote Data Literacy: Provide training and resources to ensure that employees understand how to interpret and use data. This includes not only data analysts but also managers and staff in non-technical roles.
Encourage Collaboration: Data analytics should not be siloed in one department. Encourage collaboration between data scientists, IT teams, and business units to align on objectives and insights.
Lead by Example: Leadership must embrace and prioritize data-driven decision-making. When executives lead with data, it encourages others in the organization to follow suit.
Ensure Data Quality and Governance
The effectiveness of data analytics largely depends on the quality of the data. Clean, accurate, and reliable data is the foundation for generating valuable insights. Without a strong data governance framework, organizations risk making decisions based on inaccurate or incomplete data.
a) Data Quality
Implement processes to ensure that the data collected is accurate, consistent, and up-to-date. This includes:
Regularly cleaning and validating data.
Removing duplicate or irrelevant data.
Standardizing data formats across the organization.
b) Data Governance
Develop and enforce data governance policies that define how data is collected, stored, accessed, and shared. This ensures that data remains secure, compliant with regulations, and used ethically.
Data Security: Implement robust cybersecurity measures to protect sensitive data from breaches.
Compliance: Ensure adherence to data privacy regulations such as GDPR, HIPAA, or CCPA, depending on your industry and geographic location.
Start with Small, Focused Projects
Rather than attempting a large-scale implementation from the start, it’s advisable to begin with small, manageable projects. This allows you to learn, test, and refine your analytics strategies without taking on too much risk.
Steps for Starting Small:
Identify a Pilot Project: Choose a specific business problem or area where data analytics can provide immediate value, such as improving customer segmentation or predicting sales trends.
Set Clear Metrics: Define success metrics to measure the effectiveness of the project. For example, you might track improvements in efficiency, cost reduction, or customer satisfaction.
Iterate and Improve: Use the insights gained from the pilot project to refine your approach. As you build experience, you can scale up analytics efforts to other areas of the organization.
Build a Skilled Analytics Team
A skilled and diverse analytics team is essential to the success of your data initiatives. The team should consist of individuals with a variety of expertise, including data scientists, analysts, business intelligence specialists, and IT professionals.
Key Roles in a Data Analytics Team:
Data Scientists: Responsible for creating advanced analytical models, predictive algorithms, and machine learning solutions.
Data Analysts: Focus on interpreting data, creating reports, and providing actionable insights to business stakeholders.
Business Intelligence (BI) Specialists: Develop dashboards and visualizations to help teams easily understand complex data.
Data Engineers: Design and manage the infrastructure required for data collection, processing, and storage.
Project Managers: Oversee the implementation of data analytics projects, ensuring they stay on track and meet business objectives.
Upskilling Existing Employees
Invest in continuous training to upskill your existing staff in data analytics. This can be done through online courses, certifications, and workshops. Empowering employees with the right skills will enhance the adoption of data analytics across your organization.
Leverage Advanced Analytics Techniques
Once basic data analytics capabilities are in place, organizations can begin exploring more advanced techniques to unlock deeper insights. These techniques, powered by artificial intelligence (AI) and machine learning (ML), enable organizations to predict future trends, automate decisions, and gain a competitive edge.
Key Advanced Analytics Techniques:
Predictive Analytics: Uses historical data to predict future outcomes, such as customer behavior, market trends, or equipment failures.
Machine Learning: Enables systems to automatically learn from data and improve performance over time without being explicitly programmed.
Natural Language Processing (NLP): Analyzes text data, such as customer feedback or social media posts, to identify trends, sentiments, and insights.
Prescriptive Analytics: Recommends actions based on predictive models and simulations, helping organizations make optimal decisions.
Monitor and Evaluate Performance
Once your data analytics strategy is in place, it’s important to continuously monitor and evaluate its performance. This will help you identify areas for improvement and ensure that analytics efforts are aligned with business objectives.
Key Performance Indicators (KPIs) to Track:
Return on Investment (ROI): Measure the financial impact of your analytics efforts.
Data Quality Metrics: Track the accuracy, completeness, and consistency of your data.
Adoption Rate: Monitor how widely data analytics tools and practices are being adopted across the organization.
Business Impact: Evaluate how data-driven insights have improved business outcomes, such as increased revenue, cost savings, or customer satisfaction.
Foster Continuous Improvement
The world of data analytics is constantly evolving, with new technologies, techniques, and trends emerging regularly. To maintain a competitive edge, organizations must foster a culture of continuous improvement. Stay updated with the latest advancements in the field, and be prepared to iterate on your analytics strategy as new opportunities arise.
Continuous Improvement Strategies:
Encourage experimentation with new tools and techniques.
Regularly review and update your analytics strategy to align with business changes.
Invest in ongoing training and development for your analytics team.
Conclusion
Implementing data analytics in an organization is a transformative process that requires careful planning, investment, and a commitment to fostering a data-driven culture. By defining clear objectives, investing in the right tools, ensuring data quality, building skilled teams, and leveraging advanced analytics techniques through initiatives like a Data Analytics Training Course in Faridabad, Noida, Mumbai, Pune, and other parts of India, organizations can unlock the full potential of their data. With continuous monitoring and improvement, data analytics can help organizations stay competitive and make more informed, impactful decisions.
Comments