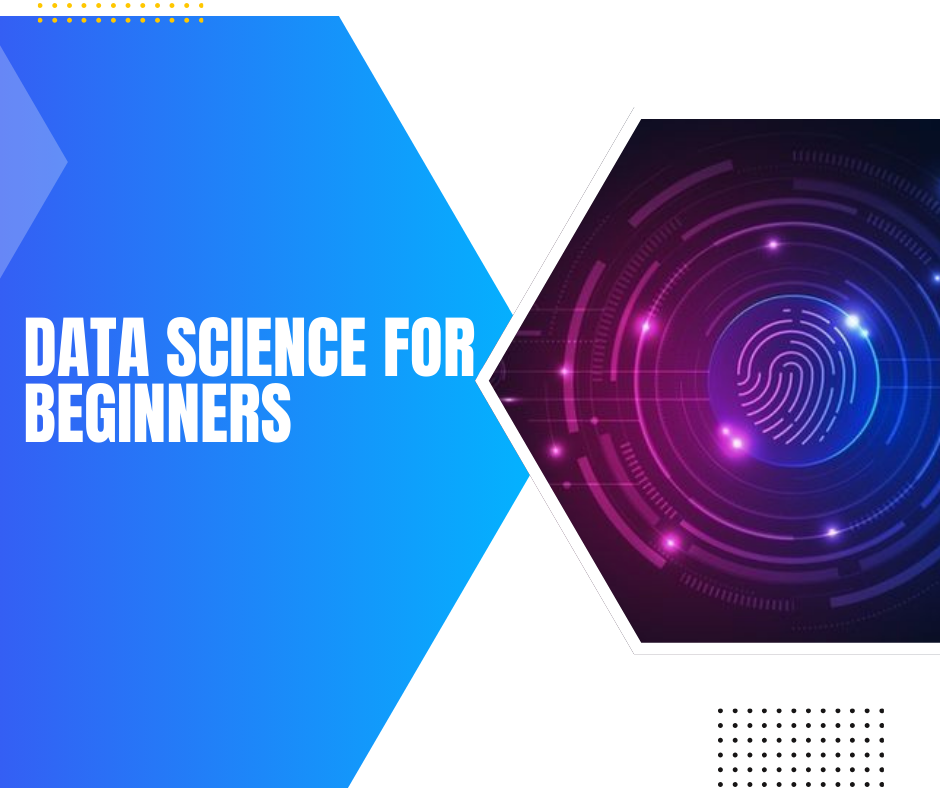
Data science has emerged as a pivotal field, driving innovations and decisions in nearly every sector of the economy. From healthcare to finance, and from marketing to logistics, data science plays a crucial role in extracting valuable insights from data, aiding in strategic decision-making. You can opt for a Best Data Science Training in Noida , Indore ,Pune, Chennai and other parts of India.
For beginners, the journey into data science can seem daunting due to its interdisciplinary nature, which blends knowledge from mathematics, statistics, computer science, and domain-specific knowledge. However, this step-by-step introduction aims to demystify the field and guide novices through the essentials of data science, offering a clear path forward for those interested in mastering this sought-after skill.
Step 1: Understanding the Basics of Data Science
Data science is essentially about extracting knowledge and insights from structured and unstructured data. It involves various stages including data collection, cleaning, exploration, modeling, and interpretation. The ultimate goal is to make informed decisions based on data. As a beginner, it's important to familiarize yourself with these stages and understand the key concepts and terminologies used in data science.
Step 2: Learning the Necessary Mathematics and Statistics
A strong foundation in mathematics and statistics is crucial for any aspiring data scientist. You don't need to become a mathematician, but a solid understanding of algebra, calculus, and statistics is necessary. Focus on statistical concepts like mean, median, mode, standard deviation, probability distributions, and hypothesis testing. These concepts are the building blocks for data analysis and predictive modeling.
Step 3: Gaining Proficiency in Programming Languages
Python and R are the most popular programming languages in the field of data science due to their simplicity and the vast array of data science libraries they offer. Python, being the more widely used of the two, is known for its readability and versatility. Start by learning the basics of one of these languages, then delve into libraries like NumPy, Pandas for data manipulation, Matplotlib and Seaborn for data visualization, and Scikit-learn for machine learning.
Step 4: Understanding Data Management Tools
Data scientists often work with large volumes of data, which necessitates the use of databases and data management tools. Familiarize yourself with SQL (Structured Query Language) for data retrieval and manipulation in relational databases. Additionally, an understanding of NoSQL databases, which are useful for working with unstructured data, can also be beneficial.
Step 5: Mastering Data Cleaning and Preprocessing
Data cleaning is a critical step in the data science process. It involves handling missing values, removing duplicates, and correcting errors in the data. This step ensures that the data is accurate and ready for analysis. Preprocessing includes techniques to transform raw data into a format that can be easily analyzed, such as normalizing or scaling numerical values, encoding categorical variables, and feature engineering.
Step 6: Exploring Data Analysis and Visualization
Data analysis and visualization are key to uncovering insights from data. Learn to use descriptive statistics to summarize data characteristics. Visualization tools and libraries like Matplotlib and Seaborn in Python help in creating graphs and charts that make it easier to understand trends, patterns, and outliers in the data.
Step 7: Diving into Machine Learning
Machine learning is at the heart of predictive modeling and decision-making in data science. Start with understanding the basics of machine learning, including supervised and unsupervised learning, classification, regression, clustering, and dimensionality reduction. Experiment with building simple models using Scikit-learn, and gradually progress to more complex algorithms.
Step 8: Practicing on Real-World Projects
The best way to learn data science is by doing. Participate in competitions on platforms like Kaggle, work on personal projects, or contribute to open-source projects. These experiences will help you apply what you've learned in real-world scenarios, build a portfolio, and gain confidence in your abilities.
Step 9: Exploring Advanced Topics
As you become more comfortable with the basics, start exploring more advanced topics in data science. This could include deep learning, natural language processing, big data technologies, and advanced machine learning models. Learning these skills will open up new possibilities and career opportunities.
Step 10: Building a Network and Continuing Education
Data science is a rapidly evolving field. Stay updated with the latest trends, tools, and technologies by reading research papers, attending workshops, and joining data science communities and forums. Networking with professionals in the field can provide valuable insights, mentorship, and job opportunities.
Conclusion
Embarking on the journey into data science can appear formidable at the outset, given its foundation in diverse disciplines like mathematics, statistics, and computer science. However, the structured approach outlined in this introduction offers beginners a manageable pathway into the field. Starting with the basics and progressively diving deeper into programming, data management, and advanced analytical techniques, novices can build a solid foundation.
Real-world application through projects and continuous learning are key to mastering data science. This field demands technical proficiency and a curious mind that thrives on solving complex problems. As data continues to play a crucial role in decision-making across industries, the skills of data scientists become ever more valuable. By committing to this path, aspiring data scientists can unlock a world of opportunities, contributing to innovations and insights that drive progress and efficiency in various sectors. The journey is challenging, yet immensely rewarding, offering a fulfilling career at the forefront of technological advancement.
Embarking on the journey into data science can appear formidable at the outset, given its foundation in diverse disciplines like mathematics, statistics, and computer science. However, the structured approach outlined in this introduction offers beginners a manageable pathway into the field. Starting with the basics and progressively diving deeper into programming, data management, and advanced analytical techniques, novices can build a solid foundation. Real-world application through projects and continuous learning are key to mastering data science.
This field not only demands technical proficiency but also a curious mind that thrives on solving complex problems. As data continues to play a crucial role in decision-making across industries, the skills of data scientists become ever more valuable. By committing to this path, aspiring data scientists can unlock a world of opportunities, contributing to innovations and insights that drive progress and efficiency in various sectors. The journey is challenging, yet immensely rewarding, offering a fulfilling career at the forefront of technological advancement.
Comentarios