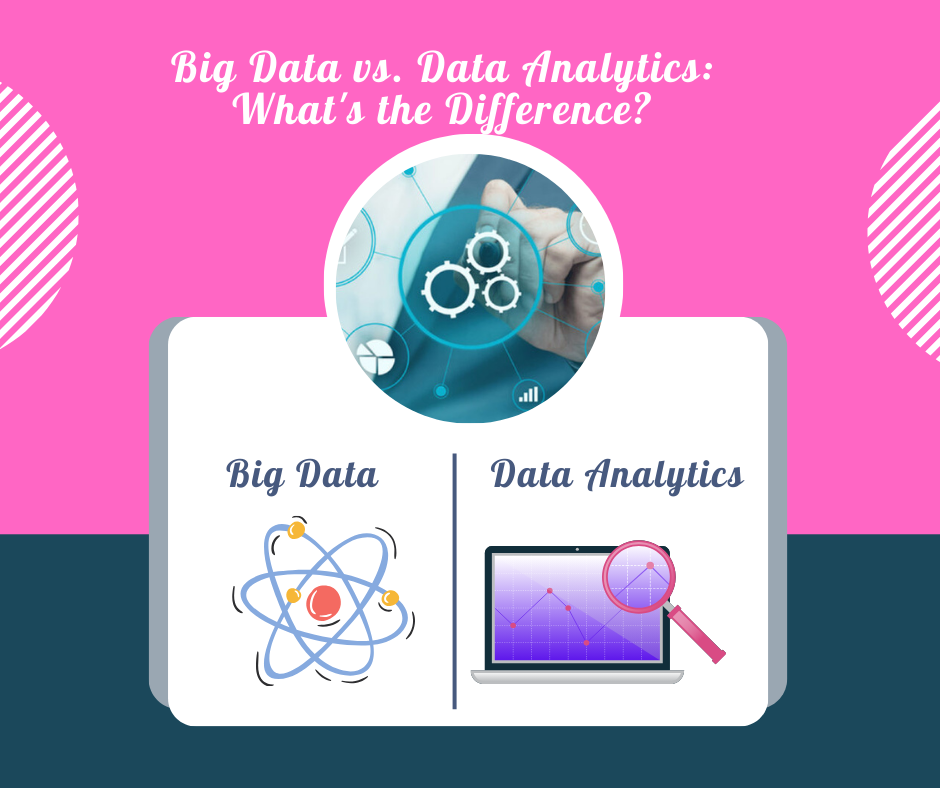
In today's fast-paced digital world, both Big Data and Data Analytics are essential to gaining valuable insights that can drive business decisions, improve efficiency, and boost innovation. However, while these terms are often used interchangeably, they represent distinct concepts and processes. This article aims to break down the differences between Big Data and Data Analytics in a way that is easy to understand.
What is Big Data?
Big Data refers to vast amounts of data that are too large or complex to be handled by conventional data-processing tools or techniques.These data sets are characterized by their volume, velocity, and variety, often referred to as the 3Vs of Big Data.
Key Characteristics of Big Data:
Volume: Refers to the vast amount of data generated every second from various sources like social media, sensors, transactional systems, and more.
Variety: Refers to the diverse types of data, including structured (databases), semi-structured (XML files), and unstructured (text, images, videos) data.
Big Data encompasses datasets that are often too large or complex for traditional tools to handle, thus requiring specialized platforms and technologies like Hadoop, Spark, or cloud computing.
What is Data Analytics?
Data Analytics is the process of examining and interpreting data to extract meaningful insights. It involves using various techniques and tools to analyze and visualize data, uncover patterns, and make informed decisions.
Types of Data Analytics:
Descriptive Analytics: Focuses on understanding past performance through historical data. It answers the "What happened?" question, summarizing past events and trends.
Diagnostic Analytics: Goes a step further and investigates why something happened. It uses data to explore the root causes of specific outcomes or events.
Predictive Analytics: Uses statistical models and machine learning to forecast future events. It answers the "What could happen?" question by predicting future trends based on historical data.
Prescriptive Analytics: Suggests possible courses of action by evaluating multiple decision-making scenarios. It answers "What should we do?" to optimize outcomes.
Data Analytics is not limited to handling large data volumes but focuses on extracting actionable insights regardless of the data size.
Key Differences Between Big Data and Data Analytics
While both Big Data and Data Analytics are essential for data-driven decision-making, they serve different purposes and require distinct approaches. Below are the major differences between the two:
1. Definition and Purpose
Big Data: Refers to the massive, complex datasets that require specialized tools for storage, management, and processing. The primary purpose of Big Data is to store and organize enormous amounts of information.
Data Analytics: Refers to the process of analyzing data to uncover trends, patterns, and insights. Its purpose is to convert raw data into meaningful conclusions that can inform decision-making.
2. Scope and Scale
Big Data: Deals with vast amounts of data that come from a variety of sources. It focuses on managing and storing the data at scale.
Data Analytics: Involves analyzing data, whether it’s small or large, to extract insights. It doesn’t require huge data sets but rather focuses on applying analytical techniques to any data set to reveal valuable information.
3. Tools and Technologies
Big Data: Requires sophisticated tools and technologies for storage, processing, and management. Examples include Hadoop, Apache Spark, NoSQL databases, and cloud platforms.
Data Analytics: Involves the use of data analysis tools and techniques like Excel, SQL, Python, R, and specialized software like Tableau, Power BI, or SAS to analyze and visualize data.
4. Outcome
Big Data: The outcome of Big Data efforts is typically data storage, management, and accessibility. The goal is to have a system capable of handling large-scale data sets.
Data Analytics: The outcome of Data Analytics is actionable insights. These insights can help businesses make informed decisions, predict trends, or optimize processes.
5. Use Cases
Big Data: Used in industries where enormous amounts of data are generated, such as e-commerce, healthcare, finance, and social media. Examples include tracking real-time customer behavior, processing financial transactions, or analyzing sensor data from IoT devices.
Data Analytics: Applied across all sectors, regardless of data size. Examples include analyzing customer feedback to improve services, predicting future sales trends, or identifying bottlenecks in a supply chain.
How Big Data and Data Analytics Work Together
While Big Data and Data Analytics are distinct concepts, they often work hand in hand. Big Data provides the raw data, while Data Analytics enables organizations to extract meaningful insights from this data.
For example, a retail company may collect massive amounts of data through online transactions, customer behavior, and social media interactions (Big Data). They would then use Data Analytics to analyze this data to understand customer preferences, predict future buying behavior, and optimize their inventory.
How Big Data Powers Data Analytics
Big Data solutions provide the necessary infrastructure to store and process the vast volumes of data that are often needed for advanced data analytics. Without the ability to handle large datasets efficiently, Data Analytics would be limited in scope and effectiveness. For those looking to gain expertise in this field, enrolling in the Best Data Analytics Training course in Noida, Delhi, Mumbai, and other parts of India can provide valuable hands-on experience and industry-relevant skills to work with Big Data and advanced analytics tools.
Conclusion
In summary, Big Data refers to the large volumes of data that need specialized tools for storage and processing, while Data Analytics refers to the process of analyzing this data to derive actionable insights. Both are crucial to modern businesses and often complement each other to drive data-driven decisions. By understanding the key differences between Big Data and Data Analytics, businesses can leverage these technologies effectively to improve operations, predict trends, and gain a competitive edge.
Also read more: The Importance of Data Analytics in Marketing
Comments